Episode 27: Looking Ahead At Customer Lifetime Value
- 0.5
- 1
- 1.25
- 1.5
- 1.75
- 2
James: Dan McCarthy, welcome to Spamming Zero. How are you this fine day?
Dan McCarthy: I'm doing well. Thanks for having me. It's a beautiful day over here.
James: Where are you at, Dan?
Dan McCarthy: I'm in Atlanta. I'm actually in the business school right now here at Goizueta.
James: Dan, I always get excited to talk to fellow marketing leaders who obsess about data almost as much as I do because that's an area that I love as well. Let's dive into this whole idea. I'd love for you to give us what is something that is top of mind for you right now when it comes to automation related to customer service? What's your take on it?
Dan McCarthy: Well, certainly, my knee- jerk reaction is to bring it back into the CLV framework. The interesting thing about automation is that it's going to entail this swapping of variable cost for fixed cost. From a CLV perspective, fixed costs are often ignored, whereas the variable costs factor directly into the contribution margin, and so for the same amount of revenue, there's an arbitrage there and CLV has to go up because you're ignoring the cost and you're taking all the credit for the benefit so it is an interesting... I don't know if you call it a gap in CLV, but it's certainly one of the things that, I think, motivates moving from something that's entirely driven by CLV to something that also incorporates what is my overhead, how might that be changing, and has that factor into whether I think it's a good idea to automate in the first place or not?
Brian: Dan, you've been looking at and studying the metric, the concept of CLV for quite some time now. Maybe it'd be good to just give some background on how you personally got into all of it, what drew you to it, and some of the insights and headway and progress that you've made in your work there.
Dan McCarthy: Yeah, it's really two things. The first was my background in finance that I'd spent. I had been a traditional coming out of undergrad finance, finance, finance. I'd gone to a hedge fund for about six years before coming back from my PhD, so being colored by that perspective, I think, was really powerful, but the second was meeting Pete Fader. He's a Wharton Marketing professor and he has spent many years studying customer lifetime value. The reason that it was a pretty powerful once you punch is because ultimately, when you think about it, what is CLV? It is NPV. It's the net present value of a customer. When you take a project finance way of thinking about an investment in the customer, it brings the finance together with the marketing and I think it helps clarify what it should be as well. See, it really was the combination of those two things.
James: What do you think brands can do to adopt some of these new metrics? For example, net present value. That's a new metric. A lot of brands probably wouldn't even know how to even get there. What's your advice to them?
Dan McCarthy: Yeah. I think it really is three things that you need. You need the right data to be able to do something with it and actually to measure it in the first place, and then you need the right definitions. There's a lot of people who have interesting suboptimal definitions for what these measures should be. Then you need the right predictive models to be able to actually bring it to life and predict it. You can have a great definition and the right data to be able to measure it, but if you're using the wrong predictive model, you're not going to have a good estimate of it, and so you really need those three things. I would say to get started, you don't need to have the A plus solution for all three of those things. Yeah, I think that, just to get CLV, the main thing that you need is your transaction log, and then just basic information about how profitable are your orders, and then some notion of how much you're spending to acquire customers, but usually when I'll see companies making their path along that journey, they'll start with you have to have a good transaction log. Most companies are going to have that, but they won't necessarily have say contribution profit down at the transaction level or super hyper detailed marketing spend data where it's properly linked to customer acquisition data. I think that that's where you want to work to, but oftentimes, you're starting with something a lot more basic than that. I think that'll allow you to get to the B plus solution, and then the hope is to really just iteratively work your way up from there. You got your CLVs, now we can think about how we can enrich the data to incorporate more touchpoints to be able to understand how CLV may vary across different segments that we're interested in or the levers that we can pull to be able to start to acquire more customers like our better ones. You can improve your predictive model moving to something that really incorporates all the different dynamics that might be going on with customer behavior at your particular company. It's that sort of thing that can help move you from B plus to A plus, but yeah, I think the key is really get started with something that's workable and then move your way up from there.
Brian: CLV is customer lifetime value, for anybody that's not with us at this point. It's one of those metrics that is a board- level metric for most companies, but it's a secondary or tertiary board metric. How much of that do you think is driven by the lack of precision that exists in it, and just the inherent understanding from both the investor side and the operator side that the methodology around it is relatively basic in most organizations, which makes it less reliable, which makes it hard to put too much weight on it?
Dan McCarthy: I almost think that the bigger issue is conflict of incentives, that when people are using it, there's a question of what you're trying to do with it. If it's a measure that you're going to put in front of your investors rather stakeholders, then there's a strong incentive to just make everything look good. You want your CAC to be small. Customer acquisition costs, sorry. We're throwing out all these acronyms inaudible they are. Yes, you want your customer acquisition costs to be low, you want your customer lifetime value to be super high, and so inevitably, you start arbitraging the assumptions for how you define the measures and you start pulling out certain expenses that really you might want to keep in. It's going to leave you with numbers, but they won't be diagnostic numbers. Ultimately, when I think of what it's supposed to be like, it's supposed to be a like doctor and this is like your health checkup and you want to know what your cholesterol is, and obviously, we all want our cholesterol to be low, but if it's high, it's high. We need to be realistic about it, or else, we can't get better and we might end up suffering from a heart attack. I think that there's a real analogy there, but if the consumer is not the right person, you're not going to try and get exactly the right answer. I think the precision's important, but even at the very beginning, if you really just want to get started, you can remove the model entirely. You can just go with purely historical figures where you say, " This is what the finite horizon value of customers has been and we're just going to focus on activity that we've already observed," and so there's no guesswork about models. It's just literally, " This is it." Obviously, we want to work up to a prediction, but the fact that you can have something that's purely historical base can make it a lot more auditable. It can just lead to more confidence if you are presenting this in front of your board members that there's nothing that's creeping into the numbers that's wrong because of the predictive model itself.
James: Brian, I would also go so far to say that I actually think that these metrics are things that investors care more about than ever right now. I do think that there's been a shift in the economy and everything like that that's going on. I think that investors care more about net retention, which in some facets, ties back to LTV or customer lifetime value, however we want to use that acronym, but ultimately, I think that more investors are looking at that more than even revenue right now as a lying indicator of growth and where they want to put their dollars or whether they're going to continue to invest. I think that's a shift that is happening and I think more brands are seeing investing dollars dry up. Especially in the D to C world, I think this is one of the reasons why this is happening. I think more investors are actually more focused on customer specific metrics. A lot of these brands haven't necessarily figured that out and that's why the dollars could potentially be drying up for them.
Brian: Mm- hmm. Dan, back in your hedge fund days and also when you are working with and speaking with investors, given the lack of a standardized formula, if you will, or the grayness and the manipulate ability that can exist inside of a company's data, how often was the strategy from that side of the table asking the company just for the raw data and then running your own calculations on it?
Dan McCarthy: Yeah, that's the ideal if it's possible. If it's a public company, then they really can't give you that data, unfortunately, but if you're under NDA or if you're in a private equity setting where you're evaluating a company and you're going to take a majority position or even acquire them outright, then oftentimes, when you get to that final stage of the diligence process, they'll open up the kimono and give you access to everything. It's at that stage where you really can remove a lot of the guess work, and they will still have their investor presentation, but you can actually reconstruct your own bottoms up view from first principles. That's a really nice thing to be able to do.
Brian: Yep. We certainly went through that process in our due diligence. I love this way of thinking about it where almost crawl, walk, run what is required to get to a B level or B plus level and that being really centered on transaction data combined with CAC and COGS data. Using that, you can basically get a look at it. Then you can look on cohorts going backwards and get at least the historical picture. When you start to look forwards and start to go from maybe crawling to walking, what are the additional pieces of data that you think are most influential and should be added next into the equation?
Dan McCarthy: The best thing that we would want to be able to have our older cohorts. It's this funny thing that, in some sense, it's the youngest cohorts that are the most important because they're the ones who are coming in right now, they're probably the ones who are most indicative of the customers you can acquire in the next quarter, and they're also the ones who are most malleable. If a customer's been with you for 10 years, they're probably pretty set in their ways and you may not need to do a whole lot with them, and it's a good thing, but it also means you can't really do stuff to greatly enhance their value, at least in terms of changing their purchase behavior. The challenge though is that those young customers are the ones that you know the least about by construction. You just acquired them so you don't really have transaction history for them in any real amount. To be able to marry up what you do have about them with behavior and maybe behavioral characteristics of older cohorts, it's just necessary and it's really, really valuable to be able to lean on those old cohorts to be able to pin down what you should expect the young cohorts to do. That's a tricky thing because if you think about it from the company's perspective, it's like, " Well, I can acquire different data sets, I can invest in my CRM system, but I can't make myself five years older to have a whole bunch of old cohorts." Yeah, there is an element that you can't really do a whole lot about that except to the extent that you do have many companies that are migrating to Shopify or migrating to some other transaction log. Sometimes, what ends up happening is you can't really link the same customer across the two different files and you end up just dumping or deleting all the old stuff. Yeah, certainly, you can invest in being able to link across transaction logs to the extent that you did some sort of a migration, but holding that aside, the rubber hits the road with that purchase data. The more older cohorts you have with it, the more you can bring the whole thing to life.
Brian: Then given that you have the older data from cohorts that go further back, when you say starting to look at behavioral data and characteristics, is what you're really looking for there... If we introduce this behavioral data point, so let's say for example, we're going to bring in customer service data and let's look at how the future purchase behavior so the lifetime value changes based on the number of service touchpoints that somebody has, and then are you looking for what are the data points that service touchpoints in this example that create very clear and distinct lines, and have a clear influence, and then those are the data points that you then commit to in your both historical measurement but also your forward predictions? Is that the train of thought?
Dan McCarthy: You can think of it in simplest terms as imagine that we have this great model for how valuable customers are. We've run it on the old customers, but then we also have this data about tier example, the service touchpoints, and it could be that in the first three months of each those customer's lifetimes, did they have good experiences or bad experiences or something like that? It could be that that ends up being predictive of the customer's value for those older cohorts all the cohorts. Then for the young cohorts, you've observed much less of their transaction data, but you also may have that same data point like, " Oh, over the first three months, these people had the good experiences and these ones did not." Then you can look back to the previous relationship between value and experience and apply that to the young cohort as well. It's that sort of thing you can do where you're leaning on these other data points that are available. If you had five years worth of transaction history, you may not even need that because you just observed so much that it all shakes out in the purchase history, but at the very beginning, you don't have that purchase history to lean on in assembly and so you need to look to whatever else might be there, including that service touchpoint data.
Brian: I do feel that if you're just going on the purchase history and you have a lot of data, then that would be a solid predictor of the future at an aggregate level in a static state, if that makes sense. Assuming that you're not investing millions of dollars into efforts to improve the business, assuming that the environmental factors around the business haven't changed, in that way, it would be predictive at an aggregate basis, but when you think about how this data and these metrics can inform the actual operating strategies inside the business, so if we more deeply understand lifetime value and we understand across all the insane amount of enterprise data that exists that these are the five behavioral data points or whatever those things are that will most heavily influence the lifetime value, then you can work against that and you can say, " Okay, we've identified that." Let's say it is service touchpoints, let's say that it is different products that are being purchased, let's say that it's involvement in community, whatever these sort of things are that create clear divisions in lifetime value looking at previous cohorts, you can almost use that to then do a journey mapping exercise and say, " Okay, these are the things that lead to the most successful customers. Let's track these as independent metrics on new cohorts that are coming in, and try and drive these new customers towards those milestones," if you will. Let's get them into a community or let's do our best to either improve the service touchpoint or make it so that it doesn't happen, or whatever those milestones are that you identify in your own data, and then you can be very actionable and you can actually improve the metric over time by knowing what the drivers are. Is that how you think about it as well?
Dan McCarthy: Yeah, and certainly, you can definitely do that. I think the keys to make sure that when you're getting those estimates, those correlates of value, if you're going to be taking action on them, that you have confidence that those relationships are causal. I think that's just the one caveat that I often give that your correlation need not be causation, and to the extent that the way that you estimated that effect of service touchpoints is that you had your product and then you had the existing solution and you randomly treat some customers but don't treat others, then that gives you really nice variation that can be exploited to be able to get an estimate of the impact of your product on customer value. Once you've got that pinned down from the older cohorts, then absolutely, I think for the young ones, you would expect that that relationship should hold because you did have valid variation that should give you confidence in causal estimates.
James: It's interesting. I think about a couple of brands actually that might have done this very thing, and I could be wrong here, but I can think about Comcast and Xfinity. They never give-
Brian: You're not going to compliment them, are you?
James: No. Here's the thing. We've moved a lot over the course of me and my wife's marriage. When you move a lot, that means that you have to transfer services of your internet because you need that, especially for me who works from home. You never get the same deal as a brand new customer. If you're like me, and trust me, I'm not the only one that has done this, there's many people that I know that do this, but they'll literally swap the account from... That's what I did with me and my wife. I became the account owner and then she became the account owner every time we moved so that we could get a good deal because they would never give the same deal to a brand new customer as they would an existing customer. You saw this in the telecommunications industry as well with Verizon Wireless and AT& T and T- Mobile. They would never give the same deal to a new line versus somebody who was an existing customer. Now, you're seeing some of this, I think, mixture of a shift a little bit that's happening with these companies. Do they have enough data now? Now, they're like, " Okay. Now, we understand it." Did it take them 10 years? Is that how long it takes.
Brian: Dan, what do you think is informing these strategies? You're decently familiar with that whole world.
Dan McCarthy: We've done a little bit of work with some telecom companies so you can't get too specific, but yeah, I would say that they have very good data that goes back very far so they've got a lot, but it is a mess to be able to piece it all together. It really is non trivial, but certainly, I think the dynamic that you're describing that older customers are, in some ways, locked in, that they're not moving around, that's a very real thing that plays out in the data. If you know that they're locked in as proxy for through the fact they've been with you for a real long time, then they don't have necessarily a major economic incentive to give a whole lot of discounts to you or deals, and so oftentimes, they won't. Certainly, you could imagine hypothetically the things that they could do. They basically do what I described a moment ago. They just say, " Well, let's just give some of these people discounts and let's not give some other people discounts assuming that they're all fairly old. Let's just look at the difference in cancellation rates or how much the people spend." I think what you would find is that there's not a whole lot of effect, but whatever the result might be, that can be then used in the future to decide whether or not to continue to give discounts to people like you, but yeah, I think given your experiences, I think you can probably guess what the answer might be.
James: Dan, not just my experiences. You've experienced that. I promise you Brian has. Everybody that has a cellphone or has internet has experienced exactly what I'm talking about.
Brian: The dynamics are so different in these monopolistic oligopolistic industries.
James: Listen to these words he's throwing at, Dan. What is-
Dan McCarthy: inaudible
Brian: In these industries where you can count on two hands the amount of companies that are there, and the barriers to entry are huge, and the government involvement is significant, you think about airlines, and telcos, and the energy companies, and even bordering into insurance, and that sort of stuff, it's just such a different dynamic. So much of the value, so much of caring about these metrics, and caring about the experience that you're giving is motivated by operating in a competitive ecosystem, and the need to truly earn and build trust and build affection from your customers versus there's two providers in town and I have to choose one of them, and one of them was already hooked up to my apartment, so I'll just do that one, and they're all just making a ton of money in the end anyways. I don't know. It just feels like they're such different dynamics and innovations
Dan McCarthy: Yeah, I was going to say, James, the experience I've had is kind of like what Brian's describing that if I had the ability to continue, I don't necessarily know that I would. I feel like it's mostly driven by are you one or two companies that are available and do you have a line into my building or house? That determines the answer. Yeah, it really is a sad state of affairs, but it also raises really interesting questions about lifetime value and how you decide what it is that you should do around it because inevitably, you can keep robbing from the piggy bank and chipping away from things that you had been providing to the customer. You yank those away, you keep that profit. If the customer has to stay, then they're going to stay. Inevitably, that will be deemed the right thing to do, but there's a question of how much you can do that before you've pushed it too far. I think Rob Markey and the folks at Bain, they'll call it bad profits, that there can be bad profits, but there's a question of on what sort of horizon do you need to look to really be able to see that that's not the right thing to do? That's a tough question, but if you have a shorter horizon, then you're almost surely going to infer that you got to keep yanking the service away from your good customers.
Brian: To the extent that these companies have the data and are operating in profit, maximizing ways rationally, I think that their actions show that at least they believe that you push it pretty far. I wonder if the barrier is social backlash or the government boundaries that are put in place. Airline is an example of where there's huge government involvement and safety is something that's a non starter sort of conversation where you can't cut corners on safety.
Dan McCarthy: Yeah. If the time span of those changes is two decades, you can't really expect any predictive model to pick it up because the predictive model, it can only be trained on the data that's available. I think the longer term effects, that would be more observable through a historical type analysis that really looks over long spans of time and is drawing associations maybe to other industries that had similar dynamics, but oftentimes, that would not be brought into the analysis that we would do. You can just see where you end up falling into this local optimum and it wouldn't really be able to tell you to do otherwise. Yeah, I'm not sure what the right solution is other than if you had some notion of how over longer spans of time customer satisfaction or lack of satisfaction has led to major disruptive change, you need to keep the customers happy above a certain level or something. Then that can serve as a baseline. Even if your model's telling you to go below that, you know you really shouldn't go there because you're entering the danger zone or something, but yeah, it's tricky.
Brian: Yeah, and that ties back to what we were talking about with those... It starts with transaction data, but then when you go deeper and you start to look at the behavioral data and characteristics, the better you understand what the drivers are of the top line numbers, the more tactful you can be, whether it's about maximizing profits and choosing which things to underinvest in or cut, or it's going the other way and it's like, " We want to invest in maximizing what the lifetime value is going to be." All of it relies on an understanding of what those drivers are.
Dan McCarthy: Yeah, I think it also really points out to say symmetry, that if you are evaluating an investment that you're looking to make to improve customer experience and you see that translate into more satisfied customers who are staying for longer, then you're in the good zone. You couldn't rob from the piggy bank there, so there's no concern about bad profits being earned. I think the opposite is where you need to think about it a bit more. I think the good zone for that would just be situations where you're pulling out expenses, but you can also see that it's not translating into lower customer satisfaction. If you can get this arbitrage where you're able to pull out the cost, it's not leading to customers being unhappy with you, it's been great. You just earned yourself an arbitrage. I think being able to work in some measure of customer sentiment, it can be useful for things like that.
James: We got to ask you one more question and it's going to not be related to customer lifetime value. I want you to just be Dan for a second. I want you to tell us about a good or bad experience that you've had when you've had to engage with customer service and what was the outcome of it?
Dan McCarthy: Well, one of the most recent examples was, this is going to be awfully specific, with Panera Bread. I've just had multiple bad experiences with their delivery service. If you place an order and you didn't do something right, they'll say that, " Oh, we're going to cancel the order on the backend and we're going to fix it," and then they don't, and there was another time again. I just had multiple issues with their delivery service. I don't know if it's that they've outsourced delivery to DoorDash and that's leading some of these issues, but there's a previous time where I'd asked for it to be delivered and I had this clear note, " You need to give me a call. I can come out to you." I waited and I was like, " Well, where the heck is my food?" No call, no nothing, said it was delivered. No idea where this was delivered because it wasn't delivered to the door, and so I had to order it again. It was very costly for Panera because actually both times, they ended up giving me a free meal, obviously. Yes, they ended up with me not being happy with their service and with them losing money, so not good all around.
James: Dan, it's been wonderful to have you on the podcast. Thank you so much.
Brian: Dan, where can people go to find more of your stuff? You're doing so much interesting stuff out there.
Dan McCarthy: Yeah, that was going to be one thing I was going to mention. Certainly, I talk a lot on LinkedIn and on Twitter. On Twitter, my handle is d_mccar, M- C- C- A- R, and then on LinkedIn, if you just look up Daniel McCarthy Emory, it should pull me up, but if you're interested in this stuff, I gab about it all the time, and yeah, built up a really nice community of people who chime in and keep me in my place when I'm wrong. Yah, so definitely connect with me there. I was going to mention too, when you talk about crawl, walk, run, you don't need to reinvent the wheel yourself. I have this company, Theta, and we do this. If you are interested in just getting off the ground, we'd love to work with you and help give you what those best definitions are, help you think about the data issues. Obviously, we live and breathe the predictive models and so we can certainly get you to run there really quickly, so I just wanted to put that out there, thetaclv. com is the website.
James: Thanks so much, Dan.
Dan McCarthy: Yep. Thank you for having me. This has been great.
Brian: Lot of fun.
James: If you've not subscribed to Spamming Zero, please do so. Give us a review. Also, reach out to Brian or I if you want to hear a topic that you haven't heard yet. We'd love to hear from you. Thanks again and we'll see you next week.
DESCRIPTION
Love geeking out about metrics, data, and Customer Lifetime Value? (I mean, that’s a good time right there ;)) Then, this week’s episode of Spamming Zero is for you!
We’re joined by Assistant Professor of Marketing at Emory University – Goizueta Business School and CLV Expert, Daniel McCarthy, who brings a whole lot of fascinating thoughts to the table.
What’s Covered?
- How Dan got into CLV - and the key lessons he’s learned so far
- Our expert’s take on automation as it relates to customer service
- Helping brands adopt new metrics
- Starting with the basics - crawl, walk, run
- Getting buy in: what these metrics mean to today’s investors
- Historical measurement & forward predictions
- Avoiding “bad profits”
- Understanding the drivers behind the data
- And more
Ready for more fantastic Spamming Zero conversations ahead? Listen, rate, and subscribe on Casted, Apple Podcast, or Google podcasts.
Today's Host
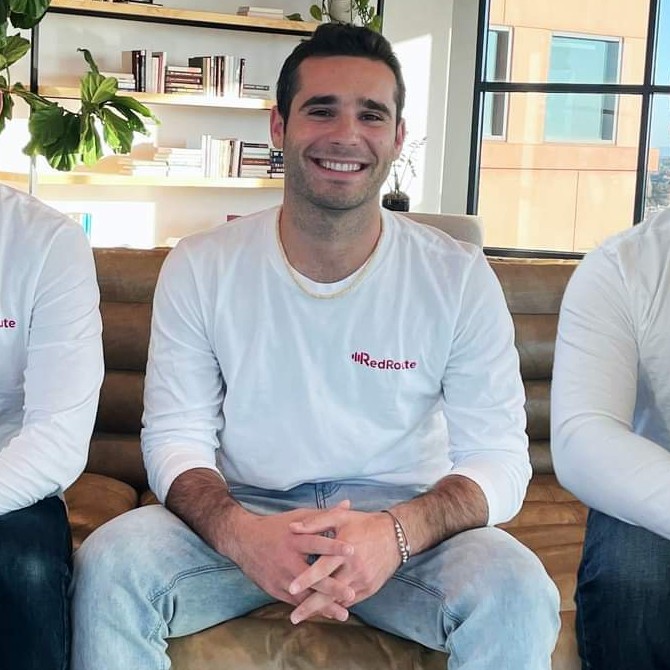
Brian Schiff
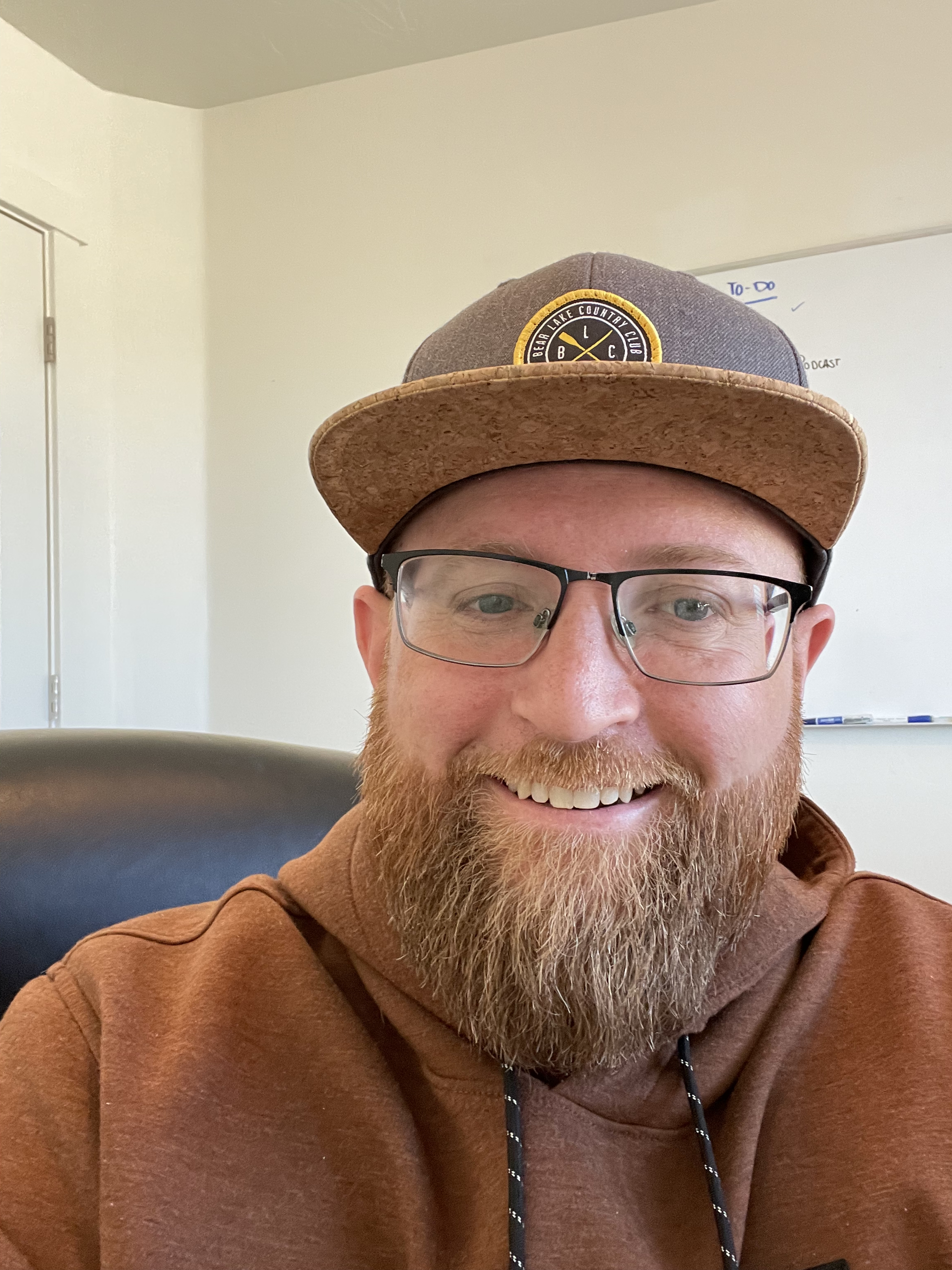
James Gilbert
Today's Guests
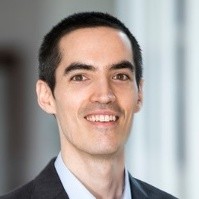