How AI can democratize data, with Clarence Lee
- 0.5
- 1
- 1.25
- 1.5
- 1.75
- 2
Speaker 4: Business school.
Daryl Pereira: Hi. Welcome everybody. This is the Business School podcast where we discuss emerging trends, what's new in the world of business. My name's Daryl Pereira. I'm a content strategist at IBM, and very excited today to be joined by Clarence Lee. And Clarence, you wear many hats. Let me turn it over to you and let you introduce yourself.
Clarence Lee: Daryl, thanks for having me today. It's such a pleasure to spend time with you. Just a little bit about myself: For the last seven years I've been a marketing faculty at Cornell's Johnson Business School. While I was there I taught a variety of marketing, product management and data science courses. My specialty in terms of research was all about applying deep learning, and one of my latest papers being one of the generative AI techniques, happy to talk about that. Specifically, I apply them to business problems. So if you think about us as marketers and applying data to various type of marketing and product issues, I basically spend most of my time thinking about how do we use data and AI to help human beings make better decisions, and these marketers and product managers make better decisions. So that was a ton of fun. How I led to that, I have this mixture of background of marketing as well as technology. So prior to that, before Cornell, I had the opportunity to get my doctorate at Harvard Business School, and I focused on customer analytics, and that's where I got a lot of my statistics and machine learning and economics chunks. I spent many years in my doctoral dissertation studying how some of the early unicorns, these startups, grow from little baby startups to a behemoth that they are nowadays in the industry, in Silicon Valley. It's fascinating to see some of the growth techniques that they were using back then. It could formalize using some of these tools in statistics and econometrics, and then eventually AI. Prior to that, before the business piece, I was a computer scientist at MIT in undergrad, so that's kind of where I got my technical foundations way back then. And so now, it almost came back to full circle to that, the whole technical roots that I have. I'm currently running a startup full- time, and I'm happy to talk about that a little bit later. But what we are doing there essentially is to try to give folks that normally don't have the technical training as we do, to have access of these very technical tools like machine learning and artificial intelligence. And so giving power to the people, that's kind of something that speaks to my heart.
Daryl Pereira: That's awesome, and as you say, quite a journey that you've been on in so many different fields that you've touched, from economics to data science to computer science. In terms of where we look at something like, for instance, the relationship between data and business, I think hopefully most of our audience here is aware of the strong interplay between the two and the importance of data to business. But can you talk a little bit about that journey and where we are right now when it comes to business and data?
Clarence Lee: That's obviously something that, it's on everybody's minds and lips nowadays with the whole explosion of ChatGPT and these large language models, the LLMs. All these developments for the last, gosh, seven months, eight months, it's been incredible; incredible, both as a consumer of these technologies, but also as a researcher that has been seeing how the field has evolved over the last 10, 15 years. So I'll go back to the basics with the data. I think whether we're talking about AI right now or basic machine learning, I think at the end of the day what people are excited about and specifically businesses are excited about is the promise of these models to help decision makers. Whether it be these product managers or brand managers who are on the ground deciding, " Hey, how do we hit the next quarter's key performance indicators," all the way to think about the CEOs, the CFOs, the CMOs discussing how do we think about big company like IBM, for instance. A Fortune 500 company that is public, and you have quarterly goals that you're being held accountable for; how do you then organize yourself as an entire organization as a unit to be able to meet certain goals? In fact, not just certain goals, but rather a whole suite and portfolio goals and then mitigating the risks. And so that's really at the end of the day, the promise of AI, this augmenting the things that we're currently trying to do and there are really hard problems that we're trying to solve. Now, we all know in order to solve that, how good these models are really depends on the data that you feed it; garbage in, garbage out. And so hence, I think if you just look even just, gosh, five years back, seven years back, there was that Economist article that talked about how we all talk about the new oil is data, and whoever controls the data, it's like controlling the oil. Basically, there's a lot of power inherently in that. And so you juxtapose that distinction in comparison to also what is also happening right now on the S& P. If you look at just the public markets, the biggest companies that are comprising of the index, the stock market, are these big tech giants. And so if you just go down the list, the alphabet, so the Googles and the Metas of the world, you look at at the end of the day, who controls and own this data, it's really these big companies. What has happened is that the market has rewarded them all the way back to the birth of these companies, way back. Think about when Google was a baby company, to them finding out their business model; when Facebook was a baby company, and then they find their business model. It was all ad supported, and they were able to basically build a empire on top of that. In order for that empire to continue running, it really depends on having access to consumer data. So if you juxtapose that and the forces of capitalism with maybe a future where we would like to go; so maybe, call me naive, call me a purist, but my dream is that eventually in the future, we'll get to a future where we as consumers would have more power and we could own and potentially make money from that data. Not so different from today when Airbnb came around, that they basically give us the opportunity to rent out our physical properties. So why shouldn't we, as generators of this data that's super valuable en masse, how do we get to a future where we as consumers have a stronger say to be able to profit from that in some ways? And then once you could figure that out, you can then let the market forces take into place. I was fortunate enough to have some economics training, so that's why I'm like, I don't really believe if just tearing that down is the solution; I do believe there should be smart regulation that's related to that. But I think the last, gosh, 40 years and 50 years in our world events and geopolitics have shown that there's this free market and these market forces, it's a force to be reckoned with. For the parts that when it works well, it works really well. And so I've spent, at least before I left Johnson, before I left Cornell, I spent a lot of time thinking about how potentially we can get from the state of things are right now where the big businesses own the data, to a future where we can liberate that and potentially give the power a little bit more to the consumer side.
Daryl Pereira: Well, that's really powerful. And just to bring that home, you did really nice job there. What you're saying is that for instance, where you look at a company like Google where a lot of its income has come through advertising, really speak- inaudible the advertising and the revenue it gets is by having a huge data pool, which is largely a data pool of us, right? Of understanding us.
Clarence Lee: Yeah, exactly.
Daryl Pereira: It provides a lot of its value. And what you're saying is that, and this gets interesting because to what degree to which then, rather than having that data pool, which is all of us, tied to one organization, what if we can start democratizing that data pool? If we can start owning our inaudible that they maybe earning, obviously each of us wouldn't earn the same amount that Google does, but individually, there could be great value in our data. So I guess there's two sides of that. One is obviously what it means for business opportunity, and the second is also what it means for us as individuals and the power that we have with that data. Would you be able to talk to both those sides?
Clarence Lee: So I think that the simplest analogy I can think about is if you think about data as property. Not so different from let's say if we think about today, do we own our home or maybe our apartment. Let's say apartment for instance. In an apartment, some of us have bigger footprints, some of us smaller footprint, square footage and things like that, but that's where we occupy our space in time. In some ways, these big companies, it's almost as if they are owning this giant apartment complex, which all of us kind of live it. We spend our days in there, and then they're able to rent that out to that space out to folks. Basically, if there's appreciation on the actual underlying property, they profit from that. And so there's that model. That's kind of the older model. But then if you begin to take a step towards homeownership; today, I own my home, I get to do whatever I want. I could rent it out, I could build a copy of it, I could basically invite friends over to share with it freely if I want. The choice is in my hands. If you think about this as slider dial, right? There's an apartment on one hand where you're renting from somebody, and then all the way on the other hand where it's, I own it, I get to rent it out and do whatever I want with it. I think if you want to transition to... like where we are now I think in the data space, is that very much that apartment. I would say just even further back from us not even owning that data. It's like, we're just leasing and then inaudible. And then where we could be as baby steps to go next is to kind of be somewhere in the middle, where it's like maybe there's a profit share that we could do with the apartment owners. You're seeing that kind of business model sometimes being played out in the real estate space. So just in the same way, I envision over the next 10 years, we can make as a market together in the data place, we can basically move from that where we are now, towards that profit sharing future. Now, the thought process then is, and sorry this is maybe a little heady, but this is how I thought about it as an academic to solve this algorithm. The question to tackle this is assuming that's where we want to go, what are the underlying forces that need to happen in order for that to happen? There's a couple of things that need to happen. One is that there needs to be a clear credit that is assignable. Whose data is it? Where does that sit? Do I sit on my device? Does it sit in the servers of the big companies itself, and all that? There's that stuff. There's also the actual business model itself. So the advertising business model that the big companies are based on; does that need to change in certain parts of that be changed and so forth? And I would say those two pieces, the solutions there are a little bit more clear. What's less clear is actually on the technology side. It's like how do we make it so that we can enable this control of flow, of data, as well as potentially sharing of data, make that a little easier. Because right now what's associated with the data, unlike real estate, is that a lot of the data, there is at the heart of it, there's this privacy issue. Today, if a company that owns our data that want to share with anybody, there is the privacy concerns that they have. If today I want to share it with somebody else, how do I not violate somebody else's privacy that I'm having with and so forth? And so getting around this privacy obstacle is something that was really fascinating to me as a researcher. I was asking myself and my team, I was like, " Assuming that the business model could work out, assuming that the behavioral changes could work out, what kind of technologies can we invent in order to enable this liberation of data?" What was really exciting to me at the same time was that I realized a few years ago that my team and I did not need to invent this technology. The technology was already invented. I just needed to see the connection between that technology that was designed for another domain and be able to rotate that to this space.
Daryl Pereira: That does really make a lot of sense. I love your analogy of this idea of ownership. It really comes down to ownership and that idea. Even thinking back to, and you touched on Airbnb and think of Airbnb versus hotels. In terms of the hotel model, we're largely consumers. But then with Airbnb and what we've seen with the sharing economies, terms like prosumer, this idea that these things get molded, and as much as where there is value in terms of if we're providing value and if the value's being handed over, why can't we have a share of that? But now you've got me tantalized on this idea of, okay, so we need this way, the privacy is very important for it to realize this goal. How can we do that, and what technology does exist?
Clarence Lee: If you think about this, the root challenge that we need to solve, is that whenever a party, party A let's say, it needs to transfer some sort of data to party B. How do we make sure that this is done in a way where it doesn't violate privacy issues? That's an example where today, hey, if I have data, I want to share it over Facebook. Or if I have data, I want to sell it to another individual or another small- and medium- sized business. How do I do that where ideally my own data, my own privacy doesn't get violated? I don't have to worry about that. Otherwise, we don't need new technology. And so, that's the, how do we have our cake and eat it too? I don't know if I had said that phrase correctly, but that's the world I was dreaming that we can get to. Now, what was fascinating is the technology that came in, I noticed, and there's a couple of different ways to do this. The technology that really, really fascinated me was, have you heard of deepfakes?
Daryl Pereira: I have, yes. In the AI space I commonly used for imagery, right?
Clarence Lee: Yeah, exactly right. So deepfakes, as we saw in the press, this must have been 2016, 2017, I can't remember the exact year. It was basically deepfakes as they are is a way to take existing videos of people and then be able to actually clone, the underlying technical term is something called data generating process. But essentially in the video space, a data generating process, it's like us right now on the video. Actual person that's emitting actual pixel values on a screen in a rectangular grid, and I could see the parts of your face that's basically corresponding to the pixel values. And these models called GANs and the technical term is generative adversarial network, they're really good at mimicking the exact configuration of pixels on a video like this, so that when you go and, once this is trained well, you can ask it to basically generate new videos based on the videos that you trained it with. So you could do that with videos. If you think about videos, videos are just really sequences, moving sequences of images. So if you could generate videos, you could generate pictures. That was a fundamental breakthrough that came out. It was super exciting in the research world because up until that point, the computer scientists, we were trying to think about what is the right way to represent knowledge? And so if you think about knowledge in a pictorial format, what's the best way to represent that in a way that does not require human checking, what they call human supervision. So this moves from supervised learning to unsupervised learning of training a machine, if you just give it a bunch of videos without saying this is a video of a cat or a dog and then this is a picture of Daryl and this is a picture of Clarence. Without that human supervision, how do we train a machine to intelligently represent that video of Daryl and video of Clarence? So that's kind of the technical description of what this is. So these GANs, essentially the underlying technology behind deepfakes, my team and I were looking at that. We're like, " Oh, wait a minute, these videos and pictures, they're just grids of numbers." And that's not so different from, if you think about the customer analytics data set that I was playing within my PhD, as well as when I'm teaching my class about growth marketing and all that. The data sets that we would generate to represent the behavior of customers, whether they come back to the app or not, do they retain? Do they churn? Do they buy certain products? If you think about that, that data is also grids of numbers, and so we came up with this idea called the picture- to- data analogy, and essentially retrofitted, the idea was very simple, we just retrofitted these GANs. We use a very basic GAN. We showed that using a very basic GAN, we could actually create high- fidelity clones of these customer analytics data. And then once we could create that, what's really interesting is if we move from just data science and then level up to let's say some sort of predicted modeling. Let's say we want to run a very basic regression that predicts whether a customer is going to churn or not and be able to predict their probabilities. Or, let's say be able to predict the probability for a segment of customer, what type of product would they buy? Is it this brand of shoes versus that brand of shoes and so forth? Very common marketing example. We found that when we train those predictive models on the real data, and then we train that separately on the what's called synthetic data that's generated from these scans, we found that it's possible to make these personalized recommendations for segments of customers without needing that actual real data. That was really fascinating to us. Because today the reason why Facebook and Google are making all that money is less so about them having your individual data, or more so is that whenever they have a new customer that comes in at any moment in time, they can make those product and page recommendations so that the consumers want to keep coming back. That's what they're making their money on. So essentially, we figure out a way where they could keep their cake and eat it, too, but then we can keep our cake and eat it, too. Because imagine today if we are able to deploy some type of generative model that could create clones, synthetic versions of our actual behavioral data, send that to Google and send that to Facebook and those big tech companies of the world. That way their business model doesn't need to change, we get to meter it, we create clones of it, and then we can potentially move towards that profit sharing version of the world in the future. And so that's what we get really excited about. That paper just came out earlier this year. Aside from that, there's also techniques like federated learning, there's techniques like homomorphic encryption, and there's polymorphic encryption and things like that. That part, I don't know as well about the security literature. But I think to me federated learning, if we could do that at scale and/ or synthetic data, we do that at scale, that holds a lot of promise in that the initial step to that world we'd like to get to. Of course this doesn't solve everything, there's a lot of problems with it, but I think that could get the world moving at a very exciting place. So I got excited for that because I see that deepfakes, it's a scary technology. I feel really mixed about it, but I'd like the fact that we can take certain technologies and potentially bring some light to the world with that technology.
Daryl Pereira: Fascinating, this idea that... Also that the problems that you're looking, because sometimes we have a, I'll say maybe it's a human condition where we might look at the problem and just disassociate it and almost just walk away from it, just along the lines of saying, " That's not great. It's not a great situation that we've got," and we might just focus on the negative aspects of it. But the degree to which you're doing, and I know I'm sure this may tie into your entrepreneurial nature as well, problems are just something that's there waiting to be solved. The fact that you then take some of these things and wrestle them by the horns. But then I think also, and as you touched on there at the end there, just the almost philosophical idea that when we see this technology, you're effectively you're using deepfake technology and you're using it in a way that can help us preserve our privacy, in a way that could then open up new business potential for us and our behavior patterns and that side of things, which feels like it ties back to the social pact we make. It is nice when I do get an ad that, if I'm looking for a car and there's an ad that's more tailored to me and in that car purchasing moment, I do prefer that to just being shown random ads about something that's like, there's still all these contracts, there's this way in which we exist. But then when we look at certainly of these technologies like the deepfake technology for instance, that has largely been covered and rightly so, in terms of it has some negative connotations in terms of what it could do in worldwide politics, in terms of what it can mean in terms of the degree to which we can create copycats, which negatively spur someone's reputation, et cetera. But you're taking that very same technology and almost looking at its essence, and then figuring out ways in which, well, actually that same technology could have a very different impact if applied in this direction.
Clarence Lee: Yeah, exactly. What's really fascinating about that, too, is when I was doing research, I realized, at least when I was even doing my PhD. The promise of a business school is that we basically have MBA students from every kind of walks of life. They could be running businesses before, they could be on Wall Street, they could be an operator or a startup or a nonprofit, or they could be a physician that comes in. One of the things that when I was being trained to become a faculty in a business school is we were being trained to kind of oversee how do we rotate these models that we would build, these predictive models that we were built, these prescriptive models that we built, across different verticals. And so what was really interesting with this exact problem that we just talked about with marketing, and we're trying to do customer churn and customer prediction, if you just change the word customer to patient, churn to let's say maybe survivability, all of a sudden now those prediction models could be actually used for the medical context, and you just change the dataset. That's something I'm super excited about, the promise of these technologies be able to at least not only be able to help people make better decisions in the for- profit space. But in the nonprofit space for instance, being able to tackle issues like improving healthcare for folks as well as climate change itself for nonprofits. That's something that really, really, it gets me excited, the why we are doing what we're doing.
Daryl Pereira: To bring that back, then, in terms of looking at your startup and where you mentioned this piece as well around talking a lot about, say, fundamental AI technology, GANs, in some of these areas; which, especially, say for smaller businesses, folks might be thinking that, " Well, okay, that's fine, but I don't have the ability to bring on board PhDs into the business. It's unsustainable." In terms of them, I know this is where your startup is focused, but what are some of the potential there that you see in terms of, it feels like some ways this might be most cases where technology can help solve a problem that it created the issue to help solve that.
Clarence Lee: So I think I want to mention a couple of things, right? There's, at the macro level, I think, what is the promise of AI and machine learning? So assuming you could get all these PhDs and all the resources, all of the money in the world; if you were to deploy it, what benefit you get from it? I think at the end of the day, the real opportunity for these models, like I said before, is augmenting humans in our decision- making. Whether you're a business owner, whether you are a nonprofit who is trying to rally a cause, maybe measurement some sort of impact that you're trying to have in your community or on the global environment, there is some outcome you're trying to shape and change about the world, and there is levers that you're dialing up and down systematically to try to impact those outcomes. And so the promise of data and AI is to be able to track that with discipline, and it makes it easier for people to essentially have superhuman level of discipline and accountability. So that's number one. Number two has to do with at a societal level. If we think about this as a nation, there are the people that have access to that discipline, that superpower, and the people that don't have a voice. How do we think about enabling and empowering the people that don't have the voice, that weren't fortunate enough to be you and I, to have the kind of education that we have, to have rubbed shoulders with the smart people that we've had the fortune to encounter with? I keep on thinking about when I was a kid, my parents, there were poor grad students. If it weren't for certain sacrifices and the things that they did to move to this country and to raise me and to go through all that grit that they had, I probably wouldn't have the privilege to go to MIT, I wouldn't have the privilege to go to Harvard and learn all this. And so what really fascinates me as I was thinking about, do I continue down this academic career or not, is I was thinking what is the most high leverage way for me to give those people that don't have the opportunities that we have that superpower? So that philosophically, that's what got me interested. I want to mention one example in the press that was super inspiring to me and my team, and that is this company called Canva. I don't know you've heard of Canva before? Daryl, have you used it before?
Daryl Pereira: I've heard. I've used it a little bit.
Clarence Lee: Apologies to them, if they're listening, the founders there. I might be butchering their mission, but what was really interesting to me when I heard about their story is, their mission was, at least when they're starting out, was to give people that don't have design superpowers, design superpowers. When they're building their next presentation, when they're building their next stationary, when they're building their next business cards, their software and their platform make it super easy so you don't have to be a designer. So then it occurred to me and my co- founder, we're like, I met them at Harvard. We're both PhDs there. We've had many years of just banging our heads against these really hard problems. And it hurts, right? Our heads are probably a little damage from that. But gee, what if we took the same exact formula that they did? But instead, instead of design as a superpower, we give people and small businesses and organizations data science and AI superpowers. How do we make it easy for them so that they don't have to be part of this inaudible this priestly class that have gone to the best schools, had the best training and can afford higher PhDs and rub shoulders with them. How do we give people that don't have that access the ability to do these things? That was a thought that I had not been able to shake off, and so that was such a strong thought that they convinced both me and my co- founder to leave our cushy tenure track positions and be like, all right, let's go stare in the scary road of entrepreneurship and just take this road that's not taken and start our company. So essentially what we do right now is we are like Canva, a canvas- like interface where you could create dashboards, you could create presentations, you could create web apps without code; and you can infuse data into it, you can infuse machine learning into it, and you can infuse what we're working on right now, generative AI techniques into it. So that's in a nutshell why we're doing it, and also what we're doing.
Daryl Pereira: That's powerful stuff. Hopefully over time, yourself, both, that feels like the message as well as what you're doing as an individual, as a business will help realize... Because it feels like there is a degree of reticence for folks to get involved in this because of the fear of the technology and with learning curve or the skill sets that you might need and the degree to it, which can be difficult. But I think I'd say also, we see this on the IBM side as well, in terms of things like the emergence of new potential in areas like low- code and no- code. Which then it feels like also this opens up, it's not just a purely economic discussion as well; because as you're pointing out, it opens it up for new areas of society, people that have largely been kept out may have the opportunity now. I think both demographically, also maybe even skills- based as well in the past, that designers and people that may be more creatively minded will have the opportunity to potentially build what in the past was largely the domain of that you had more of that kind of logical way of thinking; meanwhile, great ideas, creativity can come from all kinds of places. So it feels like that opens up quite a vista in many different directions.
Clarence Lee: Gosh, and it's been an interesting adventure, too, Darryl, to your point about creativity and surprises. One of the things I wouldn't have expected was for us to get traction in the nonprofit world. So for instance, just shout out to Reverend Leo Woodberry listening out there. He's one of the first groups that... They have their own nonprofits, and they're specifically what he was trying to do. He was trying to look out for his flock in South Carolina, where there's certain communities that are disproportionately impacted by climate change. And so he wanted a way to be able to measure the amount of that impact and also the distribution. What would that look like? Which type of households are it impacted more? Which households are not? inaudible. And so, because his community did not have that data science training, they couldn't tell their story in a data- grounded away. But with Eisengard, and this is one of those things where he was touting this in another podcast where it was just very rewarding to be able to hear how just even in beta, they were able to use our technology to impact real lives, and that was really rewarding. This is one of those things where when I was a faculty, it was really the most rewarding thing for me was the people that I had the privilege of teaching. So my PhD students that I got to see from baby researchers to become full- grown faculty themselves; to my MBA students, my undergrad students where they were thinking about their careers. But the people that I was impacting and had the privilege of dealing with, they're very privileged. To be able to go to Cornell and to afford the tuition and all that. But with Reverend Woodbury and the other customers that we have, this takes it to another level. These are demographics that I never had a chance to be able to help before, and then we could do that at scale. That's really been one of the most reporting parts of this journey, this entrepreneurial journey so far.
Daryl Pereira: That's really cool. I know we could go on, this discussion could keep on going, but I need to do my due diligence and start rounding us home. In terms of if somebody wants to find out more about you and your work and what you're interested in, and then to follow up with you, how can they get in touch with you?
Clarence Lee: The easiest way? Just find me on LinkedIn. It's just Clarence Lee, and then my email, it's just Clarence, C- L- A- R- E- N- C- E, @ eisengard. ai, and that's E-I-S- E- N- G- A- R- D.
Daryl Pereira: Wonderful. We'll share all that information. You'll find it in the show notes together with this podcast episode. Just remains for me to thank you, Clarence. This has been eyeopening. We hear a lot about this, the interplay of business data, AI, to see how that all comes together; but more importantly then the degree to which this can be opened up for a broad number of people where you ended there, in terms of some of the democratization possibilities here. This definitely gives me goosebumps. So I thank you, appreciate you taking the time out to talk with us today, and it just remains me to say this has been the Business Schooled podcast. Keep tuned, look out for future episodes or past episodes, where we discussed some of the emerging trends and what's happening in business. So stay tuned. Thanks, Clarence.
DESCRIPTION
Clarence Lee is a digital marketing and data science expert who has taught at Cornell University and is currently launching an AI startup. In this first of a series of episodes exploring the impact of AI on business, Clarence explains how AI can give consumers back power over their data, and how it is used in marketing.
Your host: Daryl Pereira, IBM Senior Content Strategist
Key topics:
- There is huge potential in AI and data to help businesses and people make better decisions. However, currently big tech companies control most of the data and benefit from it.
- AI technologies like generative adversarial networks (GANs) could allow individuals to generate synthetic versions of their data to preserve privacy, and enable a future where individuals get compensated for their data.
- Clarence’s startup Eisengard.ai aims to make AI/ML more accessible to small businesses and nonprofits, not just big companies with PhDs.
Connect with Clarence on LinkedIn
You can learn more from Clarence Lee in his Marketing AI, and Growth Marketing certificate programs offered at a deep discount through eCornell — they are great for anyone interested in leveraging AI and data in their marketing strategy.
Today's Host
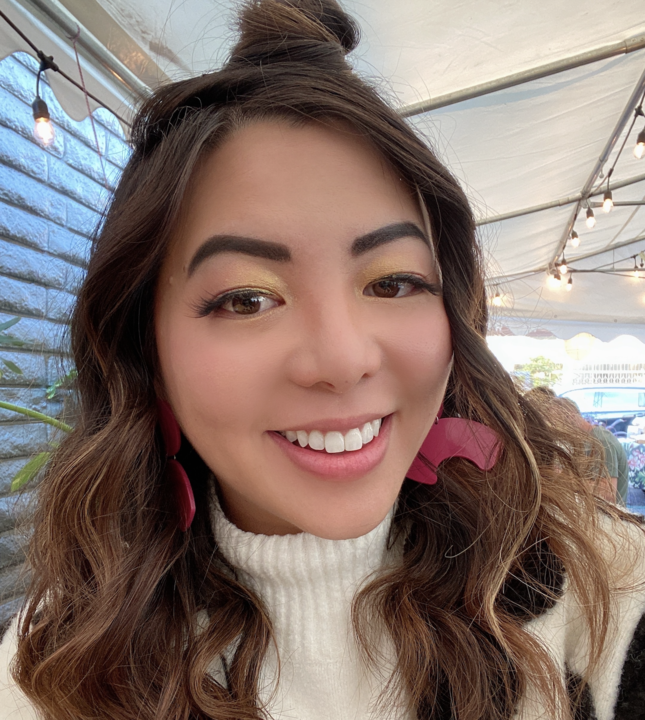
Cristina McComic
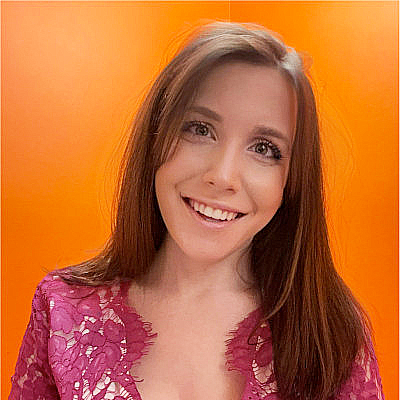
Sam Smitte
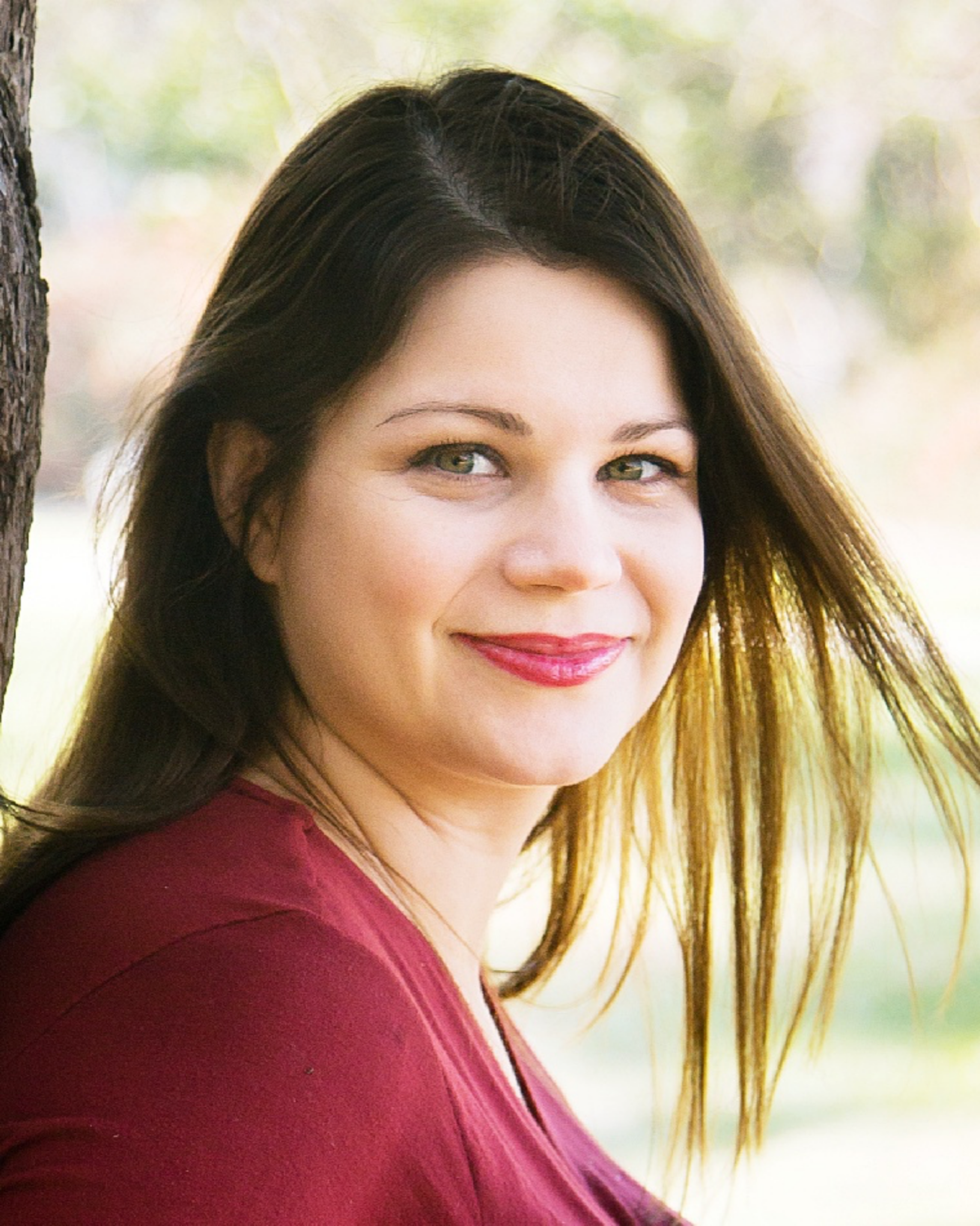
Amanda Downie
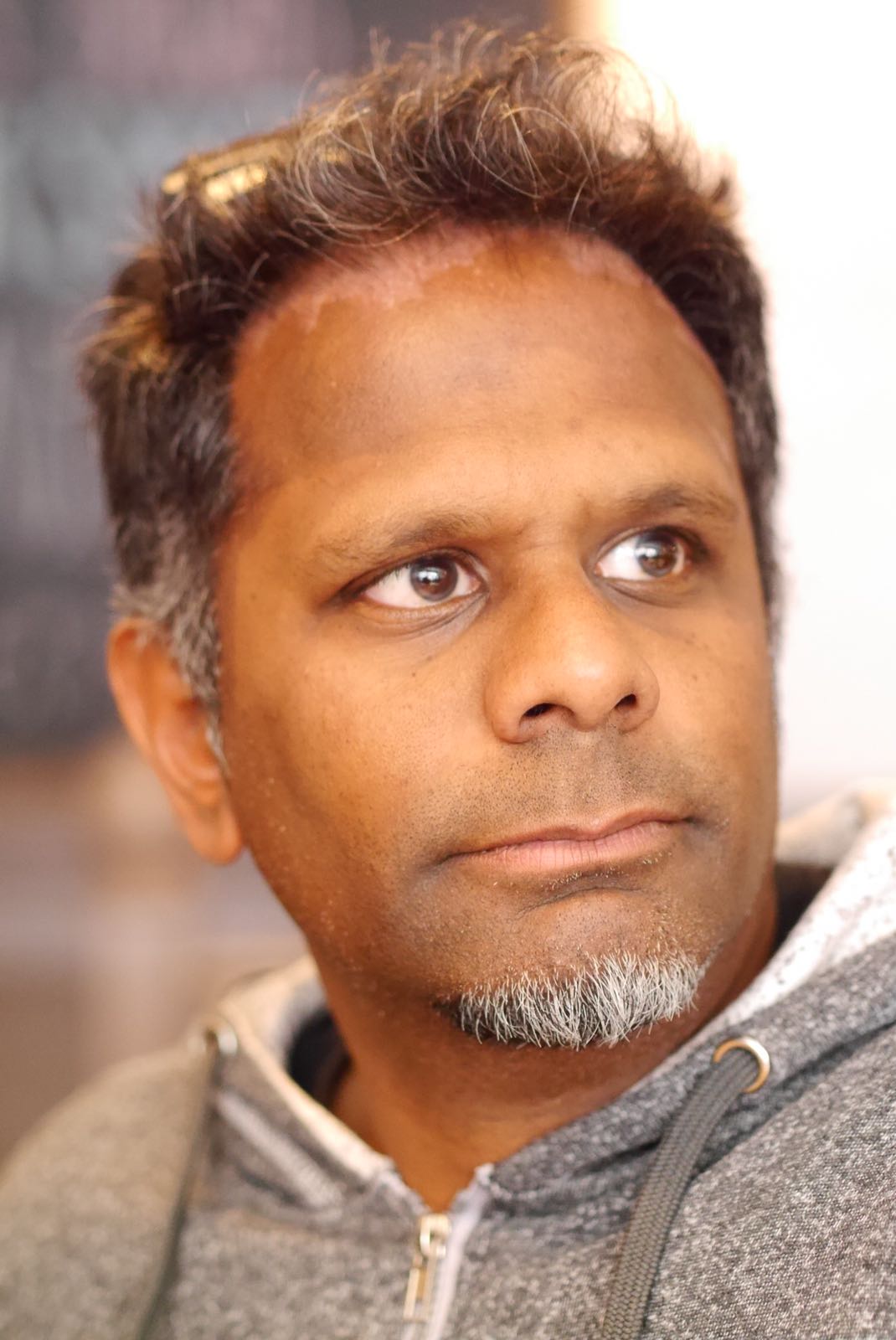
Daryl Pereira
Today's Guests
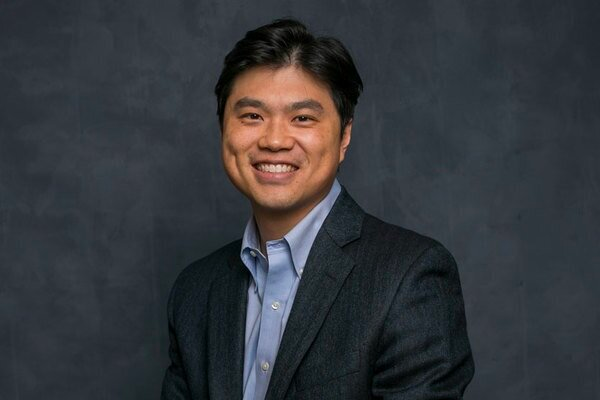