Artificial Intelligence in Tradecraft
- 0.5
- 1
- 1.25
- 1.5
- 1.75
- 2
Announcer: Welcome to the World of Intelligence, a podcast for you to discover the latest analysis of global military and security trends within the open source defense intelligence community. Now onto the episode with your host, Harry Kemsley.
Harry Kemsley: Hello and welcome to this edition of Janes' World of Intelligence. Your host Harry Kemsley and as usual, my co- host, Sean Corbett. Hi, Sean.
Sean Corbett: Hello, Harry.
Harry Kemsley: So we have spoken a great deal over the previous podcast episodes about a number of things, not least the introduction of artificial intelligence and other advanced technologies into the world of intelligence. And I thought today we might spread a little bit more of our understanding into what's happening in the AI world by bringing a real expert in. And I'm delighted to welcome Martin Keene. Hello, Martin.
Martin Keene: Hey, Harry. Hey, Sean. Yeah, my name is Martin Keene. I'm an IBM master inventor, is kind of my job title. And I work quite a lot in AI, particularly sort of presenting AI topics and teaching them through our training.
Harry Kemsley: Yeah, very good. And Martin's far too modest to tell us that he's also got an incredible number of patents to his name for the work that he's done. I believe the number's in the hundreds, is it not Martin?
Martin Keene: I think it just crossed over 400, yeah.
Harry Kemsley: 400 patents. Good Lord. Anyway, so delighted to have you with us. As you are aware from our earlier conversation, Martin, the focus of this podcast is essentially around how we derive value from the open source environment for intelligence purposes. We have spoken a great deal about how the tradecraft of the open source analyst is one that we at Janes certainly sent a great deal at time perfecting. But we also recognize that the advent of advanced technology, including things like artificial intelligence, is transforming or potentially transforming it. So that's where we want to try and center the conversation today. In and around the tradecraft and how AI is forcing change. And as we do so, recognizing that there are limits, there are factors to consider, and so on. So what I want to do today is focus on that. To get us started, given your expertise, given your background in the AI environment, perhaps you could just give us a quick summary on what are the latest things that we're thinking about in terms of AI applications and models? And then we'll start to pivot that into the world of defense more directly. So what's going on in the world of AI?
Martin Keene: So what we're seeing is really two paths in the world of AI, specifically in the area of generative AI. So I think we're all familiar with what generative AI is. And we can think of it as text- based large language models producing text output, but it's so much more than that. And we'll talk today about some of the use cases of how generative AI has actually been used for real- world situations. And it can really advance our co- intelligence. The AI becomes our co- intelligence through this. And it's taking two paths. So there's the frontier model side. So these are the big models that we've all heard of. So the GPT-IV model for example. Then Anthropic have their own model Claude, Google have their own model Gemini, and so forth. These are the big frontier models. At the same time, there's a set of generative AI models coming through the open source community, which are much smaller in terms of parameter size. Which is how we measure the size of and complexity of a model, but are rapidly catching up the capabilities of these frontier models. And because they're open source models, the weights are available. They're things that we can run in- house, and we can modify them and make changes to create our own version of these models as well. So that's really the big thing going in AI right now, is this difference between the large models and then these open source models, all producing generative AI models. And the capabilities are just absolutely moving so quickly right now.
Harry Kemsley: When you look at the changes over the last three or four years, what I see as a layperson is that AI appears to have come out of nowhere. Suddenly everywhere... If you don't have the letters AI in your workflow somewhere, somehow you're not doing it right. There's just AI everywhere. To what extent is AI, however, actually as pervasive as it seems? Or is it actually nowhere near as pervasive yet?
Martin Keene: Well, AI has certainly not come out of nowhere. Generative AI has come out of nowhere. This ability for large language models, that is something that is new that came about with the transformer architecture that Google introduced. But we've been using AI in our daily lives for all sorts of things, for image recognition on our phones and all sorts of things like that. It's been available. But yes, we're seeing it in an extremely pervasive way right now, and it feels like it's being used even when perhaps it didn't need to be used. Right now, everyone is trying to cram a generative AI capability into their app or to their website or to their offering, into their internal business processes as well. So yes, I think from the outside, if you've not been following the progress of AI and then suddenly you're introduced to generative AI, it may kind of feel like it has come out nowhere. But it's been around for a while.
Harry Kemsley: I think that's probably a symptom all I was alluding to there, which is if you haven't got AI somewhere in your business, somewhere in your work, you're really not that relevant. You're a bit old school... Sean?
Sean Corbett: Yeah, no, I was just bring it into the relevance for the intelligence analyst. And absolutely Martin, as you say, AI has been used for a while. But I think in terms of the traditional AI, if you want to call it that, that the analyst is used to using it. It's just managing large quantities of information. And the one thing that really intelligence analysts struggle with is huge amounts of data that needs to be sorted, filtered, and the relevant stuff extracted from it. And that's kind of almost getting into the mindset now, the analyst, it is a tool to be used on this. What I think that we're going to talk on about, unless I've got this badly wrong, is the generative AI. It takes the next level in terms of providing solutions to complex problems. So it's what I know as in the intelligence world, as the" so what and what if." Now at that stage, the analyst, including myself, start to get really nervous. Because is that saying that the analyst can then be replaced by AI up to a point? And hopefully we'll talk about when the person comes in the loop, do they need to come in the loop? Will that actually make things worse? But I think that's the area that is the fascinating one and where people see both the opportunity and the risk.
Harry Kemsley: Yeah, I agree. So Martin, in that regard then, it's that explainability. You and I have spoken about this before in November, we were both there. But I seem to recall a question about whether we really need to explain how AI is working in the black box. Because we don't know how some, I think somebody used the example of anti- skid breaks. We don't know how it works. We press the brake and it works. Why do we need to explain the content? If you remember that discussion that was had then. How much of what the intelligence analyst needs to understand is available to understand in AI?
Martin Keene: Yeah, this is a really fascinating area that's seen a bit of progress very recently. It is called interpretability. So yeah, this is addressing the fact that the AI kind of works like a black box. So if we think about a traditional program, how it works, it's a series of logical instructions. And if something goes wrong, that's a bug and we can figure out why it went wrong by tracing the way through the logic. And we can say, " Oh, this is what happened," and we can fix it. But AI's models, they're not programmed, they're trained. They're trained on data, so we don't have that ability to debug them and to understand why an AI model output what it did. Except we're starting to understand that now through interpretability. Now, a really good example of this is Anthropic just recently published a paper. So they took one of their large language models, the Claude Sonnet model, and they monitored when they input certain requests, which part of the model would kind of light up. And they isolated that into millions of different what they called features. So you can see, that if you ask the model about a particular topic, which features in the model lit up. Now once they did that, that's an ability to start to open up that black box a little bit. And what they did is they took one of those features and they amplified it. Which is to say that the model would provide more weight on that feature than it previously would. And the feature they amplified was the Golden Gate Bridge. So by doing that, they figured out which part of the model was lit up every time the Golden Gate Bridge was processed by the model, and then amplified it. So every time you queried the model, it would always think about the Golden Gate Bridge. It would kind of get obsessed by it. So if you took the Claude Sonnet model prior to that amplification, and you asked it a question like, " What is your physical form?" It would say, " I don't have a physical form," and" I'm an AI model." But if you just amplified that function for the Golden Gate Bridge and you asked it, " What is your physical form?" it would come back and say, " I am the Golden Gate Bridge. I'm a beautiful bridge in San Francisco, blah, blah, blah." And every question you put to it would somehow relate the Golden Gate Bridge to it. So it would say, for example, somebody asked this model, " Give me a recipe for spaghetti and meatballs," and it starts listing out the ingredients for spaghetti and meatballs. But then it can't help itself. By the end, it's saying, " I would also include the Golden Gate Bridge for wonderful views while you eat this food," and so forth. So there is work being done now in really understanding the interpretability of these models so we can start to more trust the output. But it's really still at the very early stages.
Sean Corbett: So for that, for me is the crux of where we're coming to in terms of the AI tradecraft, if you like. Because in the intelligence community, what we have to do is kind of part of a definition of tradecraft and analytical standards... Is we've got to be able to show a repeatable, auditable process says, " How did you come to that conclusion or to that assessment?" And it doesn't mean to say that it's facts, but it means to say that, " Well, okay. Based on this information that I have here, I have weighted it to say this is what I think it means." Now, it is a great example that you just given. Because, and it's almost back to the" garbage in, garbage out," isn't it? How do we ensure that the generative AI is considering everything and coming up with a non- biased balanced perspective?
Martin Keene: Yeah, absolutely. And a lot of that comes down to the training data. I mean, this is the food that AI models are built on, is getting access to high quality data for the AI to train on. Because as you say, garbage in, garbage out. If you provide it with low quality data or not enough data, the output, it's always going to come up with something, but it's not going to be a high quality output. And moving this into the defense sector, we've looked at a few examples actually of where that's been the case. So for example, the US Air Force. If you look at some of their technicians, they spend something like 80% of their time looking for information when they're trying to execute a service order. So they built an AI assistant called the equipment maintenance assistant, which is able to get answers to all of these questions through natural language by querying some of that data. So a lot of good AI comes down to data management practices, being able to get hold of the right data, the quality data, and feeding that into the model.
Harry Kemsley: Yeah, I'd really like to spend just a moment more talking about the ability to start to understand what AI is doing. Because to Sean's point, I think if I'm asking AI, I'm not going to start using the letters AI... If I'm asking the black box, the machine, the technology to help me find something, I get it. It either finds it or it doesn't. It finds a relevant and accurate answer, or it doesn't. From a database of engineering content, I can probably assess that on site. Probably. What I think becomes more interesting though is when it starts to do things that are more predictive, more uncertain, where I don't necessarily have a really good reference point as why I'm asking the question. If I could see the Ais... In quotes, if I could see the" thinking" behind it. Sean's point, if I could audit it. And you described a way that that's starting to be broken open, the black box is starting to broke open. That to me, I think is something worth exploring further. Because that starts to tell me that if you could understand how the AI reaches into certain features of its understanding of things and which bits it included and which bits it didn't include, you could begin to get an assessment of the quality of the intelligence analysis. So to the extent that that's possible, do you think it is actually possible where you get AI to actually start scoring itself? Or another AI engine scoring the answer of another AI system? Is that possible?
Martin Keene: Yes. So you can get any large language model to score itself. And the score it will assign, it'll come up with something, come up with a scale that you want, and it will score itself on that scale. And it will be a complete hallucination, because the AI does not know how it came up with the response that it did, necessarily. It is no more aware of it than we are. So yes, from that perspective, just asking the model to score itself is possible, but it's not useful.
Harry Kemsley: It's not helpful.
Martin Keene: Right, not helpful at all. But an area where this could be a little bit more useful is a generative adversarial network. These are called GANs, and these are actually two neural networks that work against each other, hence" adversarial" in the name. There's a generator and a discriminator. Now, the classic problem this is used for is for image recognition. To determine, is an image correct or is it a fake image? And what this will do is, the generator will create an image or whatever it is we are trying to assess, and then the discriminator will evaluate it. And the discriminator's job is to say, is this real or was this generated? So the generator creates something, the discriminator scores it, and then the discriminator is told was it right or not. And the generator is told that did the discriminator guess right or not. This performs a feedback loop. So we go back and forth, where the generator is getting better or better at generating synthetic data, the fake data. And the discriminator is getting better and better at discriminating between the synthetic stuff and the real stuff. And that way between the two, the models are basically co- training each other to try to score each other in a more accurate way. So things like GAN could be an area that will help with this.
Sean Corbett: So that's almost counterintuitive for us because... I agree absolutely, because doing it now. If we look at an image, is it the image that we're being told it is, and there has been disinformation where, " Oh, yeah. You can see this dreadful thing has happened here," when actually it's an image from a different time, different place. And then there's the fake imagery as well. So if we can identify that as a real positive, it's the disinformation piece. I'm misinforming to an extent, but main disinformation. But if the programs then learning to hide that, then we're almost going against ourselves. Because it's learned to obfuscate the fact that it's a fake. Do you see what I'm saying?
Harry Kemsley: Unless you're looking at it though from a perspective of saying, " I'm using this generator to generate better and better AI," which means the discriminator's got to get better and better, ? But I'm actually really tricking the discriminator."
Sean Corbett: Exactly. Filtering that. Yeah.
Martin Keene: Right. But yeah, this is an example. It can be used for good and it can be used for not good. Right?
Sean Corbett: Indeed. And that comes down to the ethics question, which we had to get onto. I knew it would. So I guess I'm not supposed to be asking the questions, but can you use AI in an ethical way? I mean, it's almost back to the, and forgive me for being really blunt, but the Asimov three laws of robotics. I can't do any harm, et cetera, et cetera, et cetera. But so for me, the ethics side is really important. Because it's something that certainly within the trusted intelligence organizations that I know in the West, ethics is a really important element, and it's being considered quite a lot. For other people, not so much. But I'm guessing it must be, from what you've said, it must be possible to train the algorithms in a certain way to only come out with ethical... deductions?
Harry Kemsley: Recommendations.
Martin Keene: That's right. So if you take a trained AI model and you just put it out to the world, it doesn't have any of that around it, and it will do whatever. So you can ask it, " How do I build a bomb?" And it'll tell you how to build a bomb. So every model that is released goes through a series of reinforcement learning by human feedback, and that is the process of testing the model and then putting constraints around it so that it will not output things that we don't want it to output. So that's typically hate speech or anything dangerous, anything self- harming, that sort of thing, confidential information. You can put all that sort of stuff and wrapper it through this second layer, this reinforcement learning by human feedback layer, to make sure that the models are performing as we want. However, like anything, you can think, you've protected the model, but there's so many jailbreaks into these things and prompt engineering attacks that you can... Or not even an attack, but just wording the prompt in a certain way to bypass some of these constraints. But yeah, it is a consideration every time a model is released.
Harry Kemsley: Martin, let's just talk for a second about the appetite from a defense audience for AI. Because what I think we're seeing is that whilst there's unquestionably interest in the application of AI in the defense setting and in the intelligence setting, that there is an increasing reticence to use models that are available to the public domain and that they can't necessarily fully understand. It's the sense of, " I can't understand what is doing, so I'm going to build my own, which I'm more likely to be able to understand." So doing it in- house rather than bringing it in from third parties. Have you seen any of that yourself in your own work?
Martin Keene: Yeah, absolutely. And I have some numbers around this. Taken from a report that IBM put out, the IBM Institute of Business Value. They surveyed 600 technology executives across 32 nations, and they were asking them different questions about how they were using AI. And this idea of moving away from commercial models to in- house models was a really compelling thing that came from this. So in 2020, about half of the defense leaders they talked to said they were heavily reliant on the private sector for AI capabilities. But by 2026, the idea is to move very much away from those commercial models to in- house stuff. And there's a couple of examples in that report. Like image analytics, for example. About 49% were using the commercial models today, but they would like to get that down to 16%. It's the same for predictive analytics, machine learning and so forth. And I think there's several reasons for that. I mean, it's partly just because of the typical adoption life cycle. So you would see a high adoption of commercial models in the considering and the evaluating phases. And then as you move into implementing and operating, typically you can now say, " Right, I've evaluated this, this was a good idea, let's build our own." But I think there's also this idea that the internal models are better suited for handling the type of data that we need to train the models on, which is typically classified or sensitive data. And the commercial models just come with this risk of exposures to the vulnerabilities, the fact that it runs off- site and all the sort of potential exploitations that we've already talked about.
Harry Kemsley: And the point that we made earlier about this explainability, the auditability of the AI black box... I think it is also currently still driving the need for human in or on the loop. Somewhere very close to the decision making process, certainly.
Martin Keene: That's right. So right now, we need to think of this as a co- intelligence, something that can assist us. And we have a human there to help with that. The thing that AI algorithms are really good at is detecting patterns and correlations that a human analyst might miss. Just because it has such a bigger picture and it has so much data that can keep in mind all at once. So we can put in all sorts of structured data like satellite imagery or sensor data and stuff like that, and then unstructured data like news reports. And then it can use predictive analytics to analyze all of that, and it can find patterns that a human analyst might not find. That said, it might not find the right ones. So we still need human analysts to then evaluate what it came back with.
Sean Corbett: And that's one of the applications certainly I was thinking about when we sort of prepped for this podcast. We did one a podcast a little while ago on the chances for a second Arab Spring. And there's so many factors that could factor into that, social, political, economic, and military as well. But there are so many factors that it would be easy to miss them. Now there is, theoretically anyway, if you train the model correctly, these were the particular circumstances that resulted in this outcome. It doesn't have to be the Arab Spring. It could be any problem. Therefore, if we see those patterns again, this is the predictability. Now, intelligence is intelligence, not information. So it doesn't matter about how accurate, how deadly right, " I'm a hundred percent sure it's happening." As long as you can articulate what the probability is and where the weaknesses are. So some of the best intelligence assessments are the lowest confidence, actually. But that's where they include the, " Okay, within the amount of information we've got, this is what we think is most likely or least likely on the rest of it." And this is where I think generative AI could possibly have a really major role in the future.
Harry Kemsley: So in terms of... Before we go onto to the predictive analytics, which has come up several times in the last few moments, let me just get to the point where we've, sort of baseline, where we think we are in terms of human engagement with AI. I think we've just agreed that human in or on the loop remains important and is likely to remain important, I think, for as long as we can't really explain what's happening inside. Or indeed, depending on the use case that we're talking about. Where do you see that changing, Martin? If you project yourself forward, do you ever see a time where we think there is enough capability that the AI doesn't need a human on the loop?
Martin Keene: Yeah. So this gets us into AGI, artificial general intelligence. Now, this is a term that you've probably heard bandied around quite a bit, AGI. I don't think there's a universal agreement on it other than to say an AGI model is as good as a human expert in every cognitive field. So we can say confidently today that there are some things an AI model can do as well as a human expert. But most things it cannot. AGI says it can do it all, every cognitive field. And the question is, when are we going to get there? When are we going to have artificial general intelligence? Now, prior to the GPT-III model, which was the model that was just before Chat GPT, the general consensus was we were about 80 years away from AGI. This is a long way off. We're nowhere near. But then Chat GPT comes up, and then the average length has moved down to something like 18 years. And then there's been so many advancements since then with the models that have come out, the foundation models that have come out. But the current consensus is somewhere around eight years. We are eight years away from AGI. Now, if you just take that and put that on a graph, there's a clear forecast error here, right? We've gone from 80 years to 18 years to eight years in the space of three years. If that forecast error continues, we are actually three years away from AGI. It's not far off, where we think we will have AI systems that are as good as human experts in every cognitive field.
Harry Kemsley: So one of the areas, the cognitive field in the human experience has been striving for as long as I can remember, probably forever, is the ability to predict the future. Predictive analytics. The ability to do analytics was the aspiration for many intelligence analysts for a long time. But always being able to see forward, to create foresight for the decision makers that their work was supporting was always the key aspiration. Indeed, to have a piece of analytical work done without some sort of forecast would be deemed an incomplete piece of work, certainly in the defense and the security arena in most cases. So where are we in terms of the achievement of this elusive predictive analytics that we can rely upon in the AI field or otherwise?
Martin Keene: Yeah. Well, where we are today is in predictive maintenance, really. So predictive forecasting of things like that, but smaller ecosystems that we can really look at all the variables and then make a prediction. So the idea of predictive maintenance is to forecast equipment failures and then to optimize your maintenance schedules to address them. So that's not looking to say where's the next warfare going to occur and in which location. But it's taking some variables that are potentially predictive and then working on them. There's a good case study that with the US Army, where they have a number of autonomous vehicles. Now, if you are driving a vehicle and you start to hear a rattle or something's not quite performing the way it is, you would mark that down and then maintenance would take a look at it. But autonomous vehicles, they don't have a person necessarily sat in these vehicles listening to here or the acceleration's a bit slower than it used to be. So the idea here is that predictive maintenance is being used for autonomous vehicles where there are no drivers to notice issues, and then provide prognostic maintenance modeling and modeling that way. So that's an area that is already being used today. I think the idea of predictive analytics more broadly is still a ways off.
Harry Kemsley: Is it reasonable to say that's because with maintenance schedules, we've got a lot of engineering experience of fixing things. We know the factors we need to measure, we know the tolerances of the engineering parts. And therefore when sensors start to detect those tolerances are being broken, we can expect there to be some sort of failure. By knowing those things, we can predict them. Whereas the prediction in a more complicated arena where you're trying to predict potentially human behavior that can be so utterly opaque to analytics, it's very, very hard. Is that the difference between what we could do today with analytics and what we aspire to do in the future?
Martin Keene: That is exactly it. So the training data for predictive maintenance for maintenance in general, it's already very well understood. And that is built into the models. And also we have the real- time data, which is also super important through sensors and so forth that make that easy. Now, if you extrapolate that to what humans are doing in the political field and so forth, we don't have that kind of real- time data necessarily. And we don't have what path to follow as clearly defined in the training data.
Harry Kemsley: Now, before we go on to the punch line, which is the use of AI in trade craft and actually forming perhaps an AI trade craft, let's just talk about this potential adversaries piece. We touched on a couple of times earlier, and Sean, you and I talked about it in the past. The likelihood that a potential adversary will have exactly the same approach to AI as an allied force is debatable. The likelihood they will be governed by the same ethics and culture as ourselves is also debatable. But let's just posit that we have a potential adversary that doesn't care about the ethics of the use of AI. And that they will release AI to get on and deliver kinetic destructive effect kill chains, fully automated and autonomous. How do you deal with that? From not a military perspective, we can talk about that separately. But in terms of AI, how do you deal with that? How do you counter that sort of thing?
Martin Keene: Yeah, it's very difficult to counter. And to counter it, you really need to understand all the different ways that it can occur, these adversarial attacks. I mean, I think the ones that are pretty obvious are things like deepfake content for misinformation, that sort of thing, right? It's very, very convincing now to be able to create a deepfake voice or even a deepfake video of somebody and put out them saying something they've never said before. But it goes much further, much deeper than that. So generative AI can really help automate vulnerability exploitation. And that's because generative AI is really, really good at generating hypotheses, evaluating those hypotheses, and then updating those hypotheses based on that evaluation. Now, to put this into an example, this is... Basically simulation to reality is a really good example of this, which is in the field of robotics. Now, there's a paper published recently by the University of Pennsylvania and the University of Texas, it's called Dr. Eureka, and it describes a robo- dog. So this is a robot dog, four legs, and it was trained to balance on a moving yoga ball. And it can balance on that yoga ball when it's walking down the street. If somebody kicks the yoga ball, it's able to adapt itself and stay on the yoga ball. And to sort of, in any environment you put it in, it's able to do it. And it was trained to do this through generative AI. It was specifically GPT-IV, the same model that's in Chat GPT, was used to train this dog to stand on this ball. And yeah, this is a field called simulation to reality. And the way it does it is it develops hypotheses, which it calls reward functions, which is a measure of success. And then domain randomization, which is to apply these parameters in the real world. And it generates thousands of hypotheses about, " If this thing happens, how should the dog react?" And it tests them in simulation, and it then evaluates those hypotheses and says, " Well, this one was right. This one should be tweaked." It generates another thousand hypotheses and keeps going like that until it is able to create the simulation that really works. And then that transfers very well to reality. Now, this was a process that was hugely task- intensive for a human, because coming up with the hypotheses, testing the hypotheses and stuff would take an awful long time. But the AI, I mean, it can come up with hypotheses all day. And that sort of area, which is great for getting your robot dog to balance on a yoga ball, might not be so good if it's applied to exposing vulnerabilities in your own IT systems.
Harry Kemsley: It sounds like we've still got a ways to go then in terms of predictive analysis, particularly where we can't find the training data, the necessary understandings of all the variables that could affect the outcome. That feels like a ways away. However, presumably with the rate of progress and the rate of improvement of advanced technology, it's not inconceivable that eventually we'll be able to start to map the key variables, even some of the most complicated scenarios. To the extent that we might actually start to get some reasonable predictions, similar predictions, that we get out of things that are much more understandable, much more available to us today. It's not that inconceivable, is it?
Martin Keene: No, exactly. And that comes back to the developing hypotheses again. That the AI is able to do that so well, it's able to evaluate how well it did in performing those hypotheses based upon evidence and then coming up with new ones. So just with that model alone, that self- learning model capability, that is where we're seeing so many advancements so quickly over the last few years.
Harry Kemsley: Yeah. All right, let me start to bring this conversation to its conclusion in the area that we really wanted to focus on at the punchline, which is AI tradecraft. I'm going to get Sean to talk about in just a second what tradecraft really means to an intelligence process. Just to give us those key milestones in the development of an intelligence insight, because that I think is what we're trying to understand how AI folds into. So Sean, I'll get you to do that just a second. Once Sean's done that, Martin, perhaps what we can start to do then is overlay on top of that, where does AI fit in? Where is AI still good, potentially better than the human? And what does that mean for the overall tradecraft process? So Sean, give us a very quick synopsis of the key milestones in intelligence tradecraft.
Sean Corbett: Yeah, sure. I mean, it's, how long's a piece of string? Because tradecraft means different things to different people. But ultimately what we're talking about is an ability to explain how you've done your business. In terms of, I mentioned before, repeatable processes that ensure the user that you've been through every process. You've analyzed every piece of data that's relevant. You filtered out the stuff that is wrong, misinformation, disinformation. You applied certain sort of analytical techniques in terms of confidence levels and done all your due diligence to come up with a" so what and a what if." And you've measured that against, " Okay, I'm not too sure of that, but this is the reason why I've done it." So it is very much a cognitive process that takes lots of variables and tries to narrow them down into something that is objective and helpful and answers a question. So effectively that is tradecraft in a nutshell, which could take three hours to explain it.
Harry Kemsley: How long have you got? How long is that piece of string? So Martin, let's try and now, as I said, overlay AI onto those fundamentals of what tradecraft are trying to do in intelligence.
Martin Keene: Right. And if we put this into AI parlance, then there are two parts to this we have to focus on. There is the training of the AI models. That is all of the data stuff that you mentioned there, Sean, of making sure that we have this quality data. Because this is the food that the AI model is going to train on. Everything that comes out of the model is going to be a direct reflection of the data that we put in. So the training time data and coming up with these good data management practices, ensuring that there's high quality data that we're feeding the model, that's the first part. The second part is then the inferencing time. This is when the model is complete. We're using it, we're querying it, we're getting responses and so forth. So at that point, that's when we're looking at the aspects that you've mentioned there about explainability, trust, and so forth. And trying to understand to what degree can we trust the model that's come out. And so adjacent to this is an area we haven't talked about yet, which is digital twins. And this is where AI meets the real world. So a digital twin is a digital representation of physical things, physical assets and systems and processes. And unlike a simulation... A simulation is a model that represents the behavior of a system over time. And it's probably a mathematical model that comes up with it. A digital twin is actually a live mirror of the physical counterparts of the real world, and it's connected. The twins are connected with each other and updated in real time. So we can test something in the digital twin to get a very good understanding of how it will work in the real world before actually deploying it in the real world. And we can get with a somewhat high degree of confidence that if the thing worked in the digital twin, it's more likely to work in the real world once we've been through that test.
Harry Kemsley: That feels like something that'd be hugely important for scenarios that you would run against certain potential adversaries and their capabilities. We're going to do that in a planning environment would be a very, very useful use of that capability, I would've thought.
Sean Corbett: Yeah, absolutely.
Harry Kemsley: So Martin, if you had one thing you wanted listeners of this podcast to recall about capable modern AI in the defense environment, particularly the defense intelligence environment, what would you want them to take away from this conversation?
Martin Keene: I only get one?
Harry Kemsley: Only because if I give you more than one, you're probably going to take the one I was going to say. Then I'd have to think of one on the spot, which I ain't doing.
Martin Keene: Yeah. I think that the main thing that I want to really emphasize is that AI... Particularly generative AI, which has been the focus of this discussion... It's there right now to a decision advantage. It is an assistant to us. It can do things, some things, quite a lot better than we can do them. But it can also do things very wrong. There are hallucinations. There are things where it doesn't understand. There are things where it doesn't have the right data to perform its task. So right now we need to think of generative AI as something that can provide decision advantage to a human. And eventually maybe it will move beyond that, but that's where we are today.
Harry Kemsley: Perfect. Sure.
Sean Corbett: I'm really pleased you said that, actually. I mean, that kind of sums it up for me. So mine's far more basic than that, but I think it talks the same thing. Is that the analyst doesn't yet need to be worried about being done out of a job. But equally, coupled with that is that denial is not a valid course of action. And we've got to learn how to use it effectively as a tool.
Harry Kemsley: Yeah, I totally agree. For me, I think it's probably the fact that you've shone a light on the fact that we are stepping towards a time where we can begin to explain how AI is working. The fact that we are just starting to understand that, the idea that we can illuminate the model and see which bits of the model are being activated by different questions. That for me, I sense, is a path to a time when auditability and explainability become a reality. Rather than what feels like a very, very black opaque box right now. So Martin, first of all, thank you. I am hugely grateful for your time. I know you're a very busy person with your hundreds of patents and thousands of things to create in your work you do. So thank you for taking the time to speak to us. If any of the listeners found any of this particularly interesting, really want to go for additional conversations around this, let us know in the usual way, send us a question. We will do our very best to cover it. But Martin, I am certain we'll want to talk to you again, so please stand by for further incoming. Thank you.
Martin Keene: Yeah, Harry and Sean, thank you so much for having me on.
Harry Kemsley: Thank you.
Announcer: Thanks for joining us this week on the World of Intelligence. Make sure to visit our website, janes. com/ podcast, or you can subscribe to the show on Apple Podcasts, Spotify, or Google Podcasts. So you'll never miss an episode.
DESCRIPTION
Harry Kemsley and Sean Corbett are joined by IBM master inventor Martin Keene to explore the impact of Artificial Intelligence (AI) on open-source intelligence. The panel discusses how AI can support tradecraft, the future of AI-driven predictive analytics, and why humans are critical in evaluating AI analysis.
Today's Host
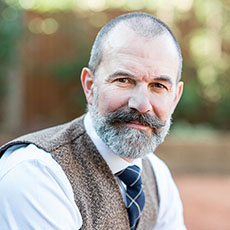
Harry Kemsley
Today's Guests
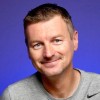