Trustworthy A.I.
- 0.5
- 1
- 1.25
- 1.5
- 1.75
- 2
Speaker 1: Why, thank you DJ and welcome all to the reboot of the Art of Automation now called the Art of AI, a podcast on trustworthy AI for business. Folks, AI is experiencing its Netscape moment, AI's been around, but now it's accessible to all. And when modern AI is paired with a human, it holds the promise of transforming the way we get work done. We become super humans and have more time to spend on the things we love. And we should also remember lessons learned from our Napster moment in the early 2000s. I'll admit it, I was caught up in that transformative peer- to- peer file sharing technology that led to the likes of Spotify and Apple Music. However, there was a systemic issue of ethics and ultimately copyright infringements, which required additional transformation in digital rights management, enabling fair business practices, which we see today. So let's kick off this series with the foundational topic of this podcast, which is trustworthy AI for business. Our guest is Ruchir Puri. He's an IBM fellow and serves as the chief scientist at IBM Research. Ruchir has been involved in the development of IBM's AI platform, Watson, dating back to its appearance on Jeopardy. He has made significant contributions to the field of AI, particularly in the areas of deep learning, natural language processing, computer vision, and of course trustworthy AI. And with that, I'd like to welcome my friend Ruchir to the Art of AI. Welcome, Ruchir.
Speaker 2: Thank you, Jerry. Wonderful to be here with the new version.
Speaker 1: Yeah, the reboot. Yeah. Thank you so much. Hey, let's get right to the questions here. Folks are eager to hear from you. Ruchir, tell our audience why are you so excited doing what you do?
Speaker 2: I've been at it almost for a decade and longer. By at it, I really mean artificial intelligence and its tremendous number of applications and exciting applications. But what I do is really AI and I think it'll be fair to say it's probably the most exciting time to be in the area because of all that is going on. When us techies start talking about stuff that's interesting to most of the word but isn't the most exciting part. It's like us nerds get excited, okay. When heads of the governments and World Economic Forum and they start talking about it, you know it has arrived actually.
Speaker 1: Yeah, when it's talk with my nieces and nephews and it's every day. It's the household word now.
Speaker 2: Exactly. And that's why I work in artificial intelligence. I've worked in it for longer than a decade and that's why I'm so excited because the potential of it is really seen by not just us techies but everyone across the society, just the human society itself and the applications of it. But just contributing to the core technology and contributing to tremendous challenges that we as a human society face and solving them is what keeps me going. Yeah,
Speaker 1: We are in a Netscape moment right now. Back in the day Netscape came out and the internet was always there, just like AI has been there, but something has happened with generative AI that now is making it more accessible to the masses, just like perhaps Netscape did back in the day. So what's your view on this recent set of activities in AI?
Speaker 2: It'll be fair to say and give credit to really likes of OpenAI and before that Google on just tremendous set of technologies that have been worked on from Google to Meta to IBM to others as well, including academic institutions like Stanford and MIT and others. When OpenAI announced ChatGPT, I would say that would be the Netscape moment. As you said, AI technology was there, but when people can touch and feel it, you don't have to go and do a sales pitch to them. Now you're just saying, Hey, they have tried it like your 10- year- old nephew may have tried it already. And they see the power of it is what is really different this time than it was in the boardroom before. It was in the client discussions before. Now it is in your living room and on your dining table actually.
Speaker 1: With that, what does it mean for AI to be trustworthy? Can you help our audience kind of think through and reason through the trustworthiness of this?
Speaker 2: If you just step back and say, where is all of this coming from? And I think it'll be fair to say what is different this time is really the massive amount of data that the latest generation of AI technologies can absorb unsupervised.
Speaker 1: Yes.
Speaker 2: Now interestingly, that is a double- edged sword as well. The reason is you can absorb all the data that you can, you can crawl the internet, but the trustworthiness of AI comes from trustworthiness of the data. Where did that data exactly come from? Well, first of all, did you have the rights to the data? So this is the first time where I see the law and the technology are really coming together in very interesting ways. I joke with some of the people, I've spent more time recently with lawyers looking than my techie friends because we want to make sure the data that we have is the data that we are absorbing legally, we are absorbing responsibly and we are attributing to it correctly as well. I think those are critical points, and I'm going to go back to the point on attribution as we discuss this a little bit further as well. So that's the first point that the governance of the data is critical to the governance and trustworthiness of AI.
Speaker 1: Okay. Data matters and the provenance of the data is a key ingredient to trustworthiness.
Speaker 2: Absolutely. Now the second part is really when you are starting to get recommendations, I would say to a large extent the latest generation of these language model or foundation models is what I'll say no next best token, what is next? And they're so good at it because they've absorbed so much of data that think about they've seen every bit known to mankind at this point in time. So they pretty much know the world around us and they can predict with amazing accuracy.
Speaker 1: Hey, Ruchir, for our Star Trek fans out there, the Borg was pretty proficient. I mean they absorbed all of the world's cultures. They can do some pretty nifty things.
Speaker 2: Yeah, exactly. Since they've seen almost everything now the question becomes, when they are giving you recommendations, can you trust those recommendations? That's the first one. This is where I weave in the point of attribution actually, which is the way you can start trusting them is if they can start telling me, by the way, this content I'm recommending you may be coming from this part of the data or of the data space. And this goes to the heart of attribution, which is attribution is needed not just for as a responsible AI, but attribution is needed as a trustworthiness aspect as well. Because I trust my colleagues and my humans at this point in time a lot more than I may trust the technology and to build trust in technology, I need to know the sources, where that data is coming from and where those recommendations are coming from. This really goes back to, I'm going to give you example of a particular technology and a product we recently worked on. It is in the domain of IT automation, what our Red Hat colleagues build called Ansible Lightspeed. Think about it this way. It helps IT automation developers sort of develop code for them and gives recommendations to them from natural language problems.
Speaker 1: Perfect.
Speaker 2: And now it's not sufficient to give those recommendations, but it is also almost required in those particular scenarios to give attribution of those recommendations as well. Because if I got a piece of snippet of a code that was written by Jerry, I would like to know Jerry authored this code because I may take it, I may modify it, I may like to contact Jerry and say, " Hey Jerry, I modified this stuff. Can you just look at it and see if it is okay in the enterprise context?" But also I may once I have a... That's why citations are so important because they build the credibility of your paper, of your project, of your proposal. And that's why I say trustworthiness of AI leads to not just the trustworthiness and the accuracy of the recommendations themselves, but also where are those recommendations lightly coming from because they're learned from the data.
Speaker 1: I see. Yeah. So for example, if you were writing an Ansible script or asking for help with a gender of AI model like Lightspeed and said, write me a script to check the status of my network router and reboot it if it's down, it's a NetApp's router and maybe it comes back with an Ansible script to do that, but it might reference the NetApp's documentation where it got that from. So if you wanted more verification or if there was a nuance or something, you have some recourse there.
Speaker 2: Exactly. So think of it as citations built into your recommendations or just like your proposal, a good written proposal will have citations on it. Think about it exactly like that. That builds a trustworthiness of it's not just sufficient to generate content, but it is also sufficient to sort of link it in the broader context as well.
Speaker 1: This is interesting, Ruchir, this sounds like something that has to be thought of from the beginning, like there's a process here involved. It's not something like once you have a model, you say, I want to make it trustworthy, you may have missed the opportunity to kind of do the right attributions from the origin of the data to the recommendation.
Speaker 2: And not just that, Jerry, I'm glad you brought this up. It needs to be baked in into the, when you are training the model itself as well. I'll give you example. When I'm trying to predict the next token or next word, let's say, I have a hundred different choices or literally millions of different choices, I would like to have next words that are more attributable. Think about it this way. So I have five choices, five very good choices. I should pick ones that are more attributable. So attribution needs to be built into the cost function of production versus as a post hoc, when you have given your... There are various ways to skin this, you've got your recommendations now you are trying to attribute versus attribution built into the design of recommendations themselves so that the content is more attributable actually.
Speaker 1: There you go. Yeah. So now Ruchir, I happen to know because we work closely together that this is not just theory. There's practice behind this. You've implemented a system with the greater IBM team and you're working this day to day. Tell us a little bit about that.
Speaker 2: So Jerry, I'm fortunate, I would say I'm blessed and fortunate to be working on these set of technologies, as I said earlier on for almost a decade. Started with the beginning of the Watson itself, going back in late 2010s and really going all the way to a more recent one than Jeopardy. And then our latest baby, I call it, growing into adult, is Watsonx. And Watsonx is IBM's foray into this area of foundation models, large language models and generative AI applied to business. This is where I think the key aspect of governance of data, trustworthiness of data, the AI that can be trusted with attributions as well, and also data that can be scaled for businesses. So data compute of AI together with its governance is three fundamental pillars of Watsonx, which is, think of it this way, what we are really trying to do is bring that B2C technology, a lot of it that exists out there in terms of OpenAI and others and people have lot of concerns, I would say, genuinely so regarding what is happening to my data? Well once I'm typing in my questions, recommendations, there is this famous case of developer not knowing enough happened to leak the secrets into the model. And since the model has learned it, now there is no recourse. And this is where I go to Jerry, you and I joke often enough on this, I call it my blender analogy, which is once a carrot goes into the smoothie that you are building, it's gone. But you can ask as much as you like, I want my carrot back, but sorry, it's gone.
Speaker 1: Oh, precious. That's my favorite analogy. I'm sorry.
Speaker 2: And this is exactly the problem, Jerry, we are trying to address.
Speaker 1: All right, so then how does Watsonx give you your bloody carrot back?
Speaker 2: So I think really, the reason is that we bring a governed state of the art with trusted data based model. Then we actually sort of think of it this way, through Watsonx enterprises can bring their data, can bring their feedback, human feedback as well, and build a model which is tuned on top of a highly qualified, highly performant pace model. That model becomes their model actually. It is not it goes into a big smoothie, which is a smoothie common to the entire world, it goes into a model which is their model now. So there is no way that can be leaked into somebody else's space at all. So by customizing it, we are actually bypassing that problem, think of it this way, if it is leaked, it's leaked to your company, it's your secrets anyway actually.
Speaker 1: So there is no one model to rule them all. We are encouraging clients to capture their unique precious data, fine tune in this curated way where you get your data, you label your data, you know your origin of your data, that's all captured. You then take that to your AI model training. You now have your AI model labeled and then you go off and you have a set of tools to govern that and life is good.
Speaker 2: At a 40, 000- foot level, that definitely is the right way to make sense of it. I would encourage people to watch IBM Research Director's, Dario Gil's keynote at IBM Think, really a phenomenal talk. I think all of you, all the audience will love it. You can go to IBM Think 2023 and go to the keynotes page and it really captures that lifecycle that you address Jerry very, very nicely there in a visual pictorial way.
Speaker 1: So if this is the state of the art in trustworthy AI, can you quickly comment on what are some of the bigger problems to be solved?
Speaker 2: I think the very first one, Jerry, I will say will be, I'll go in the order where I think it's the most important to probably harder problems as well. Number one, hallucination. Interestingly, if you look at these models, if they don't know, they'll cook things up. It's not like they'll neatly say, I don't know, they'll actually... Interestingly. I was with someone in the morning and they said, " I'm looking at this model and I typed in a question, what's the difference between a chicken egg and a cow egg?" And it really gave elaborate description of what does a cow egg look like, it's characteristics, and no human will do that obviously just cook things up. And then it really was very convincing in its description and when pushed further say, oh, I'm sorry, I was wrong. So they have a tendency to, when they don't know... Intelligence is about, you know what you know, but it's more important to know what you don't know.
Speaker 1: That's right. Are these neural networks wired or is it the end user application that is compelled to provide an answer?
Speaker 2: So I would say, Jerry, there is some fundamental work to be done in the case of models themselves as well, so that they hallucinate less. In fact, there's some wonderful work out of MIT recently just yesterday with Josh Tenenbaum and team on what is... Marvin Minsky's work on The theory of minds. And it's really based on that and it's looks into the how to reduce hallucination. So there's some very fundamental work to be done learning from cognitive science applying to neural network on how to reduce these hallucinations. Theoretical work actually. Second is in the absence of the breakthroughs, while those breakthroughs arrive, there is work to be done on solutions level, which is, I'm not trying to solve... Like take Ansible example that we were discussing before. I'm not trying to solve every problem known to mankind, which is I'm not trying to write poems, I'm not trying to do create pictures. I'm not trying to really create answer emails. I'm trying to solve Ansible, one task and one task only, which is where the problem becomes more manageable. Let me put it this way, where you can start doing things more at a solution level to correct some of the mistakes done. So think of it as a filter and a post- process because I'm doing only one task, the task is more manageable and containable. So longer term I believe, Jerry, it should be addressed at the model level and the work is going on currently in research community, but in the absence of that shorter term, from a domain point of view, that work should be and is being done at a solution level because a task sort of constraints it and contains it.
Speaker 1: So would it be fair, this would be the equivalent of a workflow within the AI engine of sorts?
Speaker 2: Yeah, yeah, absolutely. In fact, I would say it's rarely the case, Jerry, that when you deploy these models within enterprise context to solve a business problem, there is a workflow. There is actually no prompt comes in, prompt gets cleaned up, prompts get engineered to be more efficient for the model. Model takes it gives you a recommendation. The recommendation gets cleaned up, a recommendation gets post crossing, then business may apply governance around it as well because it is within a context. I know this is Ansible code, it's not just some generic tokens, words, then I can clean it up and apply some rules to it as well. And then this is a complete workflow.
Speaker 1: All right, thank you so much, Ruchir, and I appreciate you joining us on this first of our reboots of Art of AI. And if you don't mind, I'm going to go and get myself a smoothie.
Speaker 2: That's wonderful. Don't ask for your carrot.
Speaker 1: Okay. I won't ask for anything back. I get it. Thank you so much, Ruchir.
Speaker 2: Thank you, Jerry.
Speaker 1: Appreciate it. All right. The Art of AI is now off and running. I've included links to many of Ruchir's references, including Watsonx and research papers in the description section of this podcast. So follow those links. We're in the era of experiencing AI, not just talking about it. So if you're inclined, get your hands dirty with a little Watsonx today. Once again, I'd like to thank Ruchir for joining me, and I'd like to also thank you all for your continued support and interest in these podcasts. This is Jerry Cuomo, IBM fellow and VP for technology at IBM. See you again on an upcoming episode.
DESCRIPTION
Welcome to the inaugural episode of the rebranded "Art of Automation" podcast, now titled "The Art of AI." Join your host, Jerry Cuomo, IBM Fellow and VP for Technology, and guest Dr. Ruchir Puri, IBM Fellow and Chief Scientist at IBM Research, as they explore the fundamental theme of this series: "Trustworthy AI for Business." Listen in as they discuss the importance of data governance, responsible AI practices, and transparent model training to ensure that AI systems earn trust and deliver reliability in the business landscape.
In this episode, Ruchir's analogy of a blender illustrates "Un-Trustworthy AI." Just as a blender turns ingredients into an unrecognizable smoothie, AI can consume data without retaining its origin. To ensure trust, proper data governance and attribution are vital. AI models should provide clear sources for their recommendations, fostering credibility and informed decision-making. Trustworthy AI combines responsible practices and transparency, empowering users to harness its potential with confidence.
Ruchir introduces IBM's pioneering watsonx platform as a data and AI platform that’s built for business. He explains that enterprises turning to AI today need access to a full technology stack that enables them to train, tune and deploy AI models, including foundation models and machine learning capabilities, across their organization with trusted data, speed, and governance - all in one place and to run across any cloud environment.
The conversation explores the challenge of reducing AI model "hallucinations," where systems generate incorrect information with unwarranted confidence. The hosts discuss ongoing research efforts to address this issue and elevate the overall accuracy of AI outputs.
This episode sets the stage for a thought-provoking series that explores AI's transformative potential for businesses while highlighting the significance of ethics and trust in shaping the AI-driven future.
Key Takeaways:
- [03:42 - 06:37] What it means for AI to be trustworthy
- [11:13 - 15:15] The IBM WatsonX Platform
- [15:25 - 18:15] Addressing AI Model hallucinations
References:
• Improving Factuality and Reasoning in Language Models through Multiagent Debate
• Watsonx; Generative AI for business by Dario Gil, THINK 2023
* Coverart was created with the assistance of DALL·E 2 by OpenAI. ** Music for the podcast created by Mind The Gap Band - Cox, Cuomo, Haberkorn, Martin, Mosakowski, and Rodriguez
Today's Host
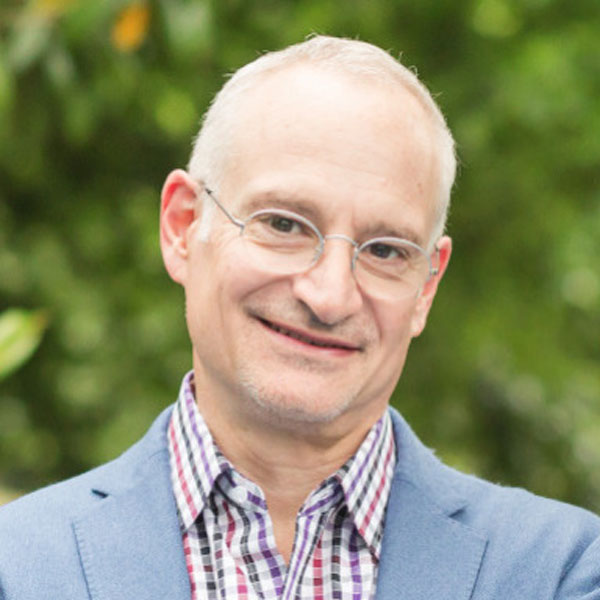
Jerry Cuomo
Today's Guests
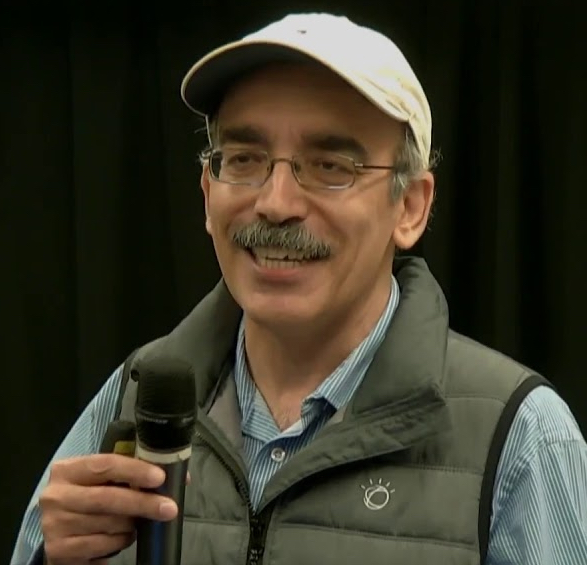