Episode 10 - Demystifying AIOps
- 0.5
- 1
- 1.25
- 1.5
- 1.75
- 2
Isabelle: Most monitoring tools can do that today. They can detect if a metric goes back to normal and then automatically cancel out or close the incident. But then the tricky piece is can you relate that with the action that you took? Because that gives you another piece of data. Next time, you can say, " Hey, I had this root cause and I applied that fix, and then the incident got resolved. Great. Next time, I might want to run this automatically."
Kevin: Hi everyone. Welcome back to another episode of The Making of the SRE inaudible podcast. Most of my guests have described the future of SRE is with AI and machine learning, having self- monitoring, self- healing, self- managing, and self- optimizing, essentially autopilot of operations. While we all appreciate and look forward to that, I have also heard from many people who are not quite sure the definition of AI, if inaudible have tasks driven by automation, is that AI or is the AI future more than that? To help us clarify AI and AIOps is Isabelle Siply. Isabelle is the STSN at IBM AIOps and she has fielded many of those questions in her journey to enable clients with AIOps. Welcome to the show, Isabelle.
Isabelle: Hello. Thanks for having me.
Kevin: So Isabelle, you must be asked this a thousand times by your customers. What is AIOps?
Isabelle: Yeah, so a AIOps, I quite like following the Gartner definition and Gartner talks about a AIOps is about combining big data and machine learning to automate IT ops processes. That includes event correlation, anomaly detection, and causality determination. I think we can expand into that as we go, but I like this. It makes it very practical because it's AI applied to a concrete problem in the area of operations.
Kevin: Thank you for the crisp definition and sounds very promising in terms of how you make the job of running a service easier. Could you please share with audience questions your clients may have asked around AIOps?
Isabelle: Very often I get this question, so I've heard AIOps and we have all of these analysts like Forester and Gartner and IDC and many others praising AIOps. So what is it like? How do I use it? How does it help me? Once we have explored that, and a lot of it from my perspective is a bit like myth busting because I feel like whenever you throw AI and people go like, " Ah, magic. Magic happens here." I think it still has the term ops in and as we all know, ops is hard work. So once we have clarified that and we can expand on that a little later, the question often is, " How do I get there? How do I get started?" I think that's a really interesting question. Maybe we pause that one for a second because you also ask, " So what's the difference between a AIOps and automation?" So I use this Gartner quote, automate IT ops processes. So there's the automation keyword, and then if you break automation down, some of the challenges that I find is it means many things to many people. If you just look at our space, automation is like a broad term across many industries, but if you look at our industry, even in the IT or telecommunication space, there is business process automation, there's a body process automation, there's CICD, there are tools like Terraform or Ansible or SALT or whatever. But in the end, I think if you net it down, automation is about reducing human intervention and process. I think that is spot on also for automation and operations. So automation I think is one option to do certain things in operations and AI helps you to do that with maybe a bit less manual effort. I quite like this quote from Carla Geiser I think is her name. She is or used to be a Google inaudible and she says, " If a human operator needs to touch your system during normal operations, you have a bug." I think that really helps. That describes nicely where AIOps and automation help in just making your systems run smoother and with less human intervention.
Kevin: That is definitely a great quote and differentiator between automation and AI. To firstly expand it, so we bust this myth and understand the magic of AIOps, can you take the audience through some use cases?
Isabelle: Let me walk you through a typical incident resolution life cycle because I think that's a good example of how and where certain AI and automation can be applied. So usually, an incident has at least five phases. So it goes from detect. You want to detect that something is wrong, ideally prior to your clients, and you need to do that over usually a pretty vast and heterogeneous set of data. The next phase is isolate. You want to find out where the issue resides and you potentially come to that complex tree of managed services ranging from infrastructure to applications. Then third phase, you want to diagnose so you understand where something broke. Now, you want to understand what's wrong. For example, are you running out of memory? Maybe you have a bug in your code or maybe you just simply did not just allocate enough memory to sustain load for this particular application. That's number three. So we had detect, isolate, and diagnose, then we have fix. Obviously, you want to do something about that problem. So you either apply a bandaid or a more permanent fix and then, in the end, you want to verify. So those are your five phases. Now, what can you do to automate that? For example, in detect, you can apply techniques to normalize your data. So it's all in the same format and schema so you can do better analysis over it. That's one way of automation. Now, you want to apply AI to it. You can, for example, automatically detect anomalies. So let's assume you are receiving a series of metrics and those metrics suddenly start deviating from a baseline. I mean, what you do usually is you set a threshold and say, " Hey, whenever my saturation exceeds a certain percentile, then please alert me." That's cool. But sometimes you might want to have metrics, you have metrics that maybe spike for good reasons and you don't want to be alerted all the time because that's false positives. So why don't you apply an algorithm that automatically detects your baseline, learns that over time, and finds out when there is actually an anomaly. For example, if a metric is flatlining, that could be perfectly okay or it could be a serious problem because you're no longer receiving something. So that's what you can do in detect. In isolate, you want to automatically identify which pieces of operational data belong together. You can do that, for example, through a series of AI and rule- driven approaches. So when I say pieces that belong together, for example, let's say you are receiving different signals from different elements in of your managed services and one signal is the root cause and the other ones are just basically errors. Obviously, you want to know the root cause. So you can either write complex rules that say, " Oh, if this event arrives on that event, and if I see this metric deviating, then please show me that as one thing," or you can do it a bit more smarter and, for example, automatically associate your operational data with the location in your services match or three or whatever you have. Then find the right boundaries in that tree of services and say, " Hey, because those are closely related or there's a service- to- service relationship between them, alert me." That can be both automation, but also AI because you can also learn sometimes what those boundaries are. So the next one is diagnose, and I've hinted at that already. So for example, say you want to detect probable cause. You can do that with all of these signals, but how do you know which one is the actual probable cause? Because it might not always be the same. You can't for sure say this event is always going to be the probable cause. So you might want to do something more clever here. Again, you can do that through a combination of techniques. So you said it, I'm part of the IBM AIOps team in particular the cloud inaudible what's in AIOps. One thing we do is what we call probable cause detection where we combine an event classifier. So we automatically taking each event and we have a pre- trained classifier and we try and flag or classify each event as either one of the golden signals and then we have two more classes. Then we also combine that with our knowledge of where that event resides in your service tree. Then to oversimplify, we would say, " Okay, an information on a top note is most likely the probable cause than, for example, an error event on a leaf node." So that's another element of combinations. The one is it's a model, it's a classifier, and the other one is an algorithm that automates that analysis. Then in moving, so we had detect, isolate, diagnose, and fix. We're now in fix. What you can do in fix is obviously, and that's a little bit moving into the holy grail. So I would say probable cause detection or suggestion plus what I'm going to talk about now, which is resolution recommendation, that gets you towards the holy grail of incident resolution. You're going to detect what it is and you want to ideally find out how to fix it. There are loads of options here and I think the industry is just getting started there because once you found out what it is, how do you find out how to fix it? That's quite challenging because there are loads of options and the more hetero genius your system is, and the broader your span of control is, the hardware gets to find the right resolution. I mean, if you're in charge of a single application or maybe a single piece of middleware, then your options might be limited. But that's fairly the case these days because our environments are growing, digital services are growing. So the breadth of what usually needs to be covered by I think operations and SRE teams out there, it's not getting smaller. So you need something that helps you, but also it increases the challenges. Verify the end to see if something is still working again. You can apply automation here as well. For example, you can, and inaudible most monitoring tools can do that today. They can detect if a metric goes back to normal and then automatically cancel out or close the incident. But then the tricky piece is can you relate that with the action that you took because that gives you another piece of data...
Kevin: Confirmation.
Isabelle: It actually helps you... Yes, confirmation. Next time, you can say, " Hey," and I'm speaking of a model that is able to learn. You can say, " Oh cool, so I had this root cause and I applied that fix and then the incident got resolved. Great, next time I might want to run this automatically." Now frankly, very little systems out there do this at the breadth that is needed because that again moves towards a really high level of automation and autonomous operations. But I think this is where we should be moving over time. It's going to be a challenge and probably stepping ahead of myself, but I think humans will always be needed in operations. I'm 100% confident we will never run fully autonomously because the space is just too complex. But we can try and reduce some of the more mundane tasks and free people up to do cool things and to improve inaudible the system, if that makes sense.
Kevin: We would all love to be doing that for sure. You captured it well, automation, we've all been through a disruption and coming out the disruption, we learn and Jerry Kumar said it really well. So that learning, we capture what we learn into code and that's what we would write into automation. I really love how he explained AI and how difference about automation is that hey, you have those hard coded learnings, but AI is able to help you think outside of the perimeters of your code, help you correlate and help you in real time solve the problem more quickly than what you may have already learned. Yeah, you started this segment by saying, the customer often asks you, " What is AIOps?" After you share with them the bright future of it, the next thing they always want to know is, " That's great, Isabelle. How do I get there?" I wonder if you can spend a few minutes on what is the approach you guide the customers to get there.
Isabelle: Yes. Let's talk a little bit about the challenges that they have because that helps in understanding how we guide them. So I see three main challenges. Number one, and that sounds a bit surprising, it's availability of data and data quality. Everybody thinks we're exploding, data volumes are exploding. We have lots of things to look at, but is it the right thing and is there enough visibility? Because I think in operations sometimes there's also a challenge of flying blind because sometimes you only know whether you have instrumented well, if you can diagnose what the cause is, when the cause is there. It's obviously some people are brilliant at this, they have tons of experience and years of learnings in their brains and they know exactly how to instrument the system, but that knowledge is rare. So there will be errors and sometimes you will only see what problem you have once it's there. So that level of data that is also processable and centrally integrable I think is a challenge as well. There is no point in flooding the system with data. It needs to be the right data because the bigger the data is, the more expensive it is. Yeah, I mean that's a standard problem. The more expensive it is to store and the harder it gets to process it properly. Challenge number two is if you want to holistically apply AIOps or techniques in that space, usually you have to cross silos. Many customers that we work with, they have fairly siloed teams. They have the networking team, the infrastructure team, their individual application teams. Getting them to collaborate for holistic analysis is not easy. That's what we need because I said we need the right data and the right data usually spans those silos. The last piece is trust. It's like with all AI projects, especially in operations where you've probably covered this in previous episodes, blame is a big thing in operations. Whose fault is it? It's hard to blame a system. So establishing trust in a system that does some things for you automatically is not exactly easy. What I usually recommend to customers is you need to look at where you have your biggest pain points, which area that you are in charge of causes you the most trouble. Then do you have access to data that might help you overcoming that trouble? Then, once you have identified that sweet spot, you can start applying some of the techniques that I mentioned and be it with an IBM product or something completely different. But as you do that, you need to take the people that are in charge with you. I'm a big believer, no operational approach can be basically imposed upon people because you have to take them with you. That's the same for AIOps because I mean, those are humans. Some people have built their career on fixing problems in production. If you tell them, " It's fine, it's going to be automated." I mean, guess what they do? I understand that. I think most of the techniques we do today, the purpose is not to take people's jobs away. It's allowing people to manage the breadth and growth that is imposed upon them through hopefully the growth of their business. I find usually, that resonates with most customers that I work with. I really enjoy it because we work across really different industries from telecommunications to banking, to travel and transport. Even though they're so different. Still, they have very similar problems when it comes to operating their businesses and services.
Kevin: I was doing reflection. I remember inaudible back. He had mentioned, I really like this analogy, when automation breaks or when AIOps breaks, you cannot say, " Hey, it was the automation's fault."
Isabelle: Yes.
Kevin: You cannot blame a piece of technology. So I think that's why people have a difficult time of trusting. You're totally right. The way to build that trust is with data, with explainability so people know and understand and appreciate what behind the scenes, the automation AI is doing. So they can trust it.
Isabelle: Agree.
Kevin: So if you had a crystal ball, could you take us to that future?
Isabelle: Yes. So hopefully it's bright because as you might have guessed from my previous sentence, I'm really passionate about operations. I love my space. I think this specific area that we're in is doing great. Very often, not as visible as it could be. As you mentioned Jerry, he asked me about a year ago, I think he said, " Isabelle, how is it that we can build self- driving cars, but cannot build self- driving operations?" You and I, we've spoken about this before and together with Kristen Stewart, a friend and colleague of mine, I've written a blog about that where we are hinting towards how this could look like. I said it previously in this conversation, I don't believe we will get to completely autonomous systems in the future because I think our world is too complex, the IT world that we're in or the telco world that we're in is too complex. But I think we can, as I said before, alleviate people from more mundane tasks, reduce some of boring repetitive work, and apply more of the advancements in AI that are very often not related to operation. When you think AI, I think most people think about autonomous driving or image recognition or speech or whatnot. Not many immediately take bridge to operations, but some of the AI elements that are in there can, I think, also be applied to operations. Because a lot in operations is also, for example, about pattern recognition. On an abstract level, you do that in images too. But even with all of that fancy AI, I think there will still be a certain amount of rigor and discipline needed in order to run businesses, in order to operate businesses. I think that will never go away. So I think the future is a combination of solid engineering work plus AI on top applied to operations.
Kevin: Definitely look forward to that. The part where I'm especially looking forward to you talk about reducing mandate task or things that's very tedious. A big part of is really learning from incidents. I think there's a lot of potential for AI to help correlate all that data. There's definitely no lack of it to help people learn from that and say, " Hey Kevin, Isabelle, if you only did this, this wouldn't have happened." Ooh, that's fantastic.
Isabelle: Yeah, I think you're touching on a great point. We haven't spoken about that, but the whole notion of learning and post- boredom feedback loops I think is super critical. Because that's something sometimes often, I mean we all notice it's often overlooked. You worked through the incident and solved. Yes. Then the next one comes around and that's no different. I technically lead a large development organization with developers over the world, like worldwide. When we fix critical things, because some of our customers complain, we don't always close the feedback loop. So if we had something that helped us doing that saying, " Hey, this is really... You should really apply this learning from this previous ticket or bug," I think that would be brilliant. I think we have loads of things we can look forward to.
Kevin: I think that may be a different episode. One of the comment to make to my team is if you ever feel like it's a dejavu, it's probably something we haven't learned yet. Ideally, learn and apply the action. I think that's important. Apply the actions of learning to it. The same type of disruption shouldn't happen again or even they did, it will have a less impact.
Isabelle: Yeah, I like that analogy.
Kevin: Called the dejavu indicator inaudible. Well, we'll work on that. So Isabelle, one of the purpose of this podcast is for technical vitality. Do you have any hints to practitioners who may look to getting into SRE on what they can do to get ready?
Isabelle: So let me start with, and I think that's true for any job, find something you enjoy and that you're curious about and then the rest follows. As soon as you start to light that fire and become passionate, learning becomes easy and becomes fun. So to me, if you want to move into SRE or operations, I would recommend to be excited and interested in it because then everything is easier. So that's my preface. Then I think there is, like many things in our industry, we're really lucky because there is a lot of publicly available free documentation. I guess you guys have already touched on some of the SRE books out there that you can read and get some foundational knowledge. Then I think you've got to get an entry level job and start training because nothing prepares you for a job in operations until you actually do it. It can be wild. It can be wild. Find an experienced mentor who has been on the job for a while and gives you those nuggets of information that aren't always in the books, like that practical, hands- on keyboard experience that helps you accelerate things. Yeah, by the way, this is not only applicable to SRE, I think it's applicable to many jobs in our industry.
Kevin: Well said. You got to have that passion to lead that fire and do that spark. So Isabelle, in closing, let's go back to the inspiration of this podcast. What would you say is your ingredient and recipe for us to embrace AIOps?
Isabelle: So I love this question as a close out of this conversation. So I think it's operational discipline and foundations like event management, correlation, anomaly detection, probable cause detection, and you combine that with some sprinkles of AI and you're well prepared, I think, for embracing the journey towards AIOps.
Kevin: I love it. Sprinkle inaudible. There you go, ladies and gentlemen, Isabelle's ingredient and recipe to embrace AIOps for SRE. Thank you, Isabelle, for coming to the show and demystify the myths of AIOps and giving a clear differentiation between automation and AI.
Isabelle: You're very welcome. I truly enjoyed this. Thanks so much, Kevin, for having me and for raising all of these interesting questions. They got me thinking.
Kevin: I'd also like to thank you all for listening. See you again on a future episode.
DESCRIPTION
Crystal ball shows that the future of SRE is with AI & ML, having self monitoring, self healing, self managing and self optimizing. Essentially autopilot of operations. While we all appreciate and look forward to that, many people are not sure of the difference between automation and AI. If we all have tasks driven by automation... is that AIOps or is the AI of the future more than that?
To help us demystify AI and AIOps is Isabell Sippli. Isabell is the STSM at IBM AIOps and she has fielded many of those questions in her journey to enable clients with AIOps.
Today's Host
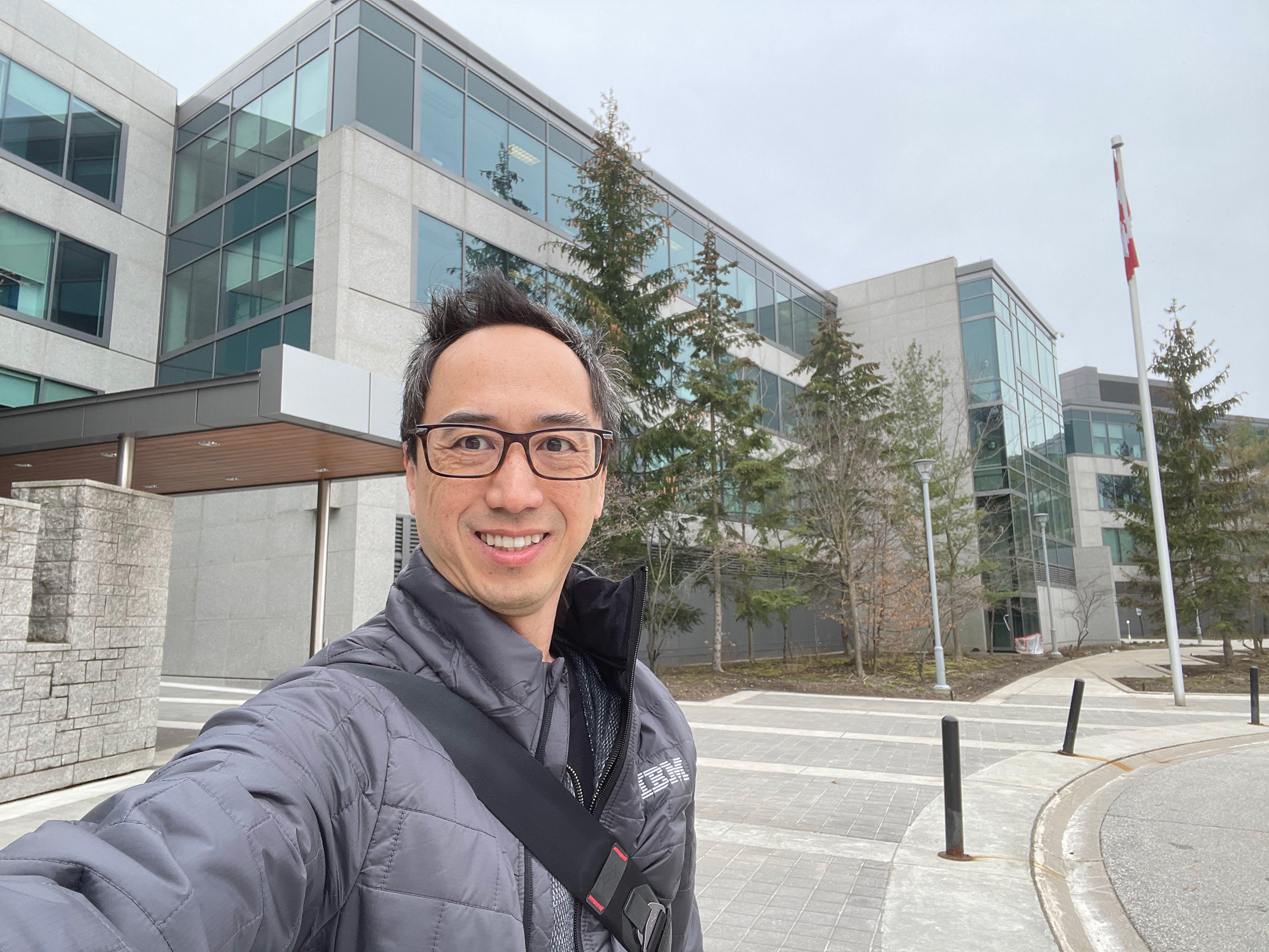
Kevin Yu
Today's Guests
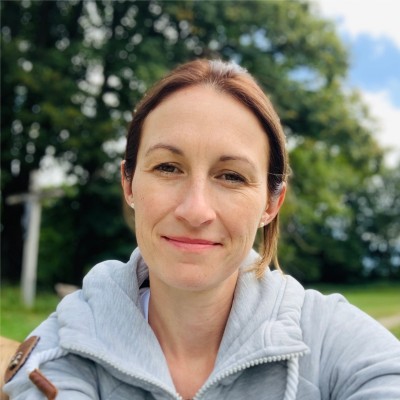