Stellar Sessions with Zach Linder and Morgan Llewellyn 🎥
- 0.5
- 1
- 1.25
- 1.5
- 1.75
- 2
Zach Linder: Hello and welcome to the first episode of Stellar Sessions. I'm your host, Zach Linder, COO of Stellar, and we're here today to talk about all the amazing things in generative AI and LLM space and activity. So my first guest on our episode is Morgan Llewellyn, our Chief Strategy Officer at Stellar who can tell us a little bit more about Stellar and then where we're going and what we need to be thinking about.
Morgan Llewellyn: Hi everyone. My name is Morgan Llewellyn. Thanks for that great intro, Zach. So Stellar is a generative AI focus company. We focus on LLMs, generative AI from a product and services perspective. And so we help organizations implement, support and maintain generative AI throughout their tech stacks. We work with small startups all the way to large organizations, government, and it's really a best of both worlds when you think about that broad reach. So we're working with small scrappy startups and we're able to apply that same scrappy and innovative approach to large organizations, your Fortune 500s, your government entities, and we're able to bring the knowledge of those large organizations down to the small startups and help them as well as they mature. And so it's an exciting time for sure right now in this space and Stellar's right in the middle of it. And yeah, I'm really excited to be here.
Zach Linder: Awesome. All right, so the world has changed in the last eight months or so and we've taken huge leaps forward. What are the things that you're most excited about and that you're looking forward to being able to use some of this new technology and capability?
Morgan Llewellyn: Well, that's a good question. That's a really good question. What am I most excited about? And that's a really hard question to answer because there's a lot to be really excited about. And the reason why I say that is there's so much opportunity for generative AI and in particular, large language models to be used throughout your business that this isn't an opportunity for a specific vertical, it's not an opportunity for a specific customer. Generative AI has applications for every organization. And why I'm really excited about LLMs in particular, just getting down to the technical nitty- gritty, besides the business impact, is LLMs are unleashing a capability that we have not seen in the data science AI, ML space over the past two decades. And it's making it accessible to people that previously weren't able to access it. And so the capability that it's unleashing is the ability to structure and organize information in a consistent and repeatable way. It's the ability to generate content for almost zero cost. It's the ability to put in an automated decision through that structure and organization of information, through the automation of content creation and through the execution. There's so many key elements that generative AI is providing today and that allows businesses to take an opportunity to take advantage of. And just summing up real quick, why it makes me personally super excited is, again, these are things that we couldn't do 10 years ago. It would've taken a ton of time for us to go and build models to understand the natural text in a specific use case of case notes for a governmental unit. It would've taken tons of time just to build that one model and then we would've had to go and figure out a different model for doing similar case notes in say an insurance company and another use case with a different organization. It would've had been so specific. But today with things like LOMs, you can spend less time building models, less time deploying those models and more time thinking about your product, more time thinking about your customer and delivering really great products.
Zach Linder: That is awesome. There's a lot to unpack there. My answer would've been, I'm really excited that I can now, through a prompt, get an image of a dog catching a Frisbee while jumping over the Eiffel Tower. That's the one answer that I would've come back with, but I like yours as well. So let's start unpacking that. So there's content generations going down to zero, which I think is really interesting. And then we've got the ability to use it across industries. And so I'm a mid- size company, I've got 50 employees, I'm in the HR, tech space, how do I use this? What are the first couple of steps I do and what are some use cases?
Morgan Llewellyn: Yeah. So we can talk about specific use cases and I think we can also talk about how do you get started? How do you think about incorporating something like a large language model into your organization? And if you're talking about HR tech in particular, or you're talking about any organization like healthcare where you've got sensitive information, PII, PHI, PCI, any of these Ps, one thing you're going to want to think about is what are the guardrails you're going to want to put around your initial use case? How do you avoid that third rail and potentially damaging situation and really stick with something safe. That would be my first suggestion. Is look, you don't have to go after the hardest problem first. There's probably a lot of value to be had with data that's more accessible and has less restrictions around its use. So that's the first thing I think about doing. The second thing I would think about doing is LLMs, generative AI, it's not about a feature. Taking advantage of these technologies is really about corporate strategy. It's really about business strategy. It's really about your product strategy. And so if you're thinking about where to take advantage of it, you want to take a strategic focus and strategic approach. One way you could do that is you can look at what does your product roadmap look like and anything that's on your product roadmap you should be considering does an LLM fit here? That's day one. That's something that you can be doing today. And why does that make sense for a product company like HR tech? It's going to be because you've already committed that this is a high priority item that we're going to go and do something around either change or net new. It's already something that you've dedicated resources to. And so you're already committed to making a change. And that change that you're going to go deploy is going to be living in your organization for the next three or five years. And so you need to be thinking about how can I incorporate an LLM? Is it applicable to what I'm working on today? And that could be a place to start.
Zach Linder: So things are changing really fast. Should I implement something now or should I wait a little bit? Do I need to wait till the next version of something that comes out? Do I need to wait for what Google's going to come out with to compete against OpenAI and Microsoft or do I deploy today?
Morgan Llewellyn: So again, if you think about your product cycle, if you're developing a new product today and you are waiting for what Google's going to come out with or what the next version of something's going to be, that decision is really around, is what is out there today good enough because you don't want to wait for that fast follow, that may never happen. And so if what's out there today is good enough, then you don't have to wait. Think about how can it be adopted today? That would be my suggestion. What do you think?
Zach Linder: Oh, I prefer to move fast every single time. So I would definitely deploy what we can today. So let's talk about a real quick example about this because you and I have been using this tool recently. So Synthesia is pretty amazing. So we built out this demo for the Rally Conference, which we're at right now in Indianapolis, Indiana. And in preparation for Booth, we wanted to make sure that we were demonstrating our capabilities. And so we were struggling with that demo. You don't want to have vaporware, you don't want to have anything that's too complex, takes a long time, you got audio issues, all your standard conference stuff. And so we went a different route. We went this AI generation route where we employed virtual people to go tell our story. And we did this with a script that we used ChatGPT to help us out with and we were able to go from zero to the one of this thing inside of two hours. So how awesome is that?
Morgan Llewellyn: So, not a sponsor. Not a sponsor, but I think that that really indicates how can generative AI be used? So think of us as a stodgy professional services organization. Or not, right? We are scrappy and we're building. We build stuff. But just consider a stodgy professional services organization who's trying to address some challenges. There are new tools out there that can do a great job that didn't exist three months ago. And I think that particular piece of tech is absolutely amazing. If you're in the startup community, you need to check this website out because if you are thinking about putting together a demo, it's an excellent product.
Zach Linder: So this ties back to the question, do I go or do I wait? What's my risk there, right? If I go now and I build a bunch of content, do I need to think about how do I manage my content differently so I can feed it to the machines or what's my strategy there? Because if I'm shifting more away from development and more around to content generation or content feeding, how do I need to think about my business and my operations?
Morgan Llewellyn: So this is a new space. Things are constantly changing. And one thing I would suggest is find someone who's done it before and avoid some of the early pitfalls that you might run into. I'm not saying that you have to go and hire a consultant. I'm not saying you have to go and hire a team to be able to do this, but being able to learn from someone who's already done this, help you avoid common pitfalls that we see all the time or help you shorten that development life cycle. That's something that all founders and Fortune 500 companies should be looking for, is someone who's done this before and can give them really good advice to avoid those common mistakes.
Zach Linder: I think that's a really good point. So I think whenever you're looking at doing something internally, let's just say you're an enterprise, you're always capacity constrained. You've got your roadmap that you've got to go deliver on, but then it's easy to go outside to get outside contractors to help. And the domain specific knowledge is also helpful, but I can go to ChatGPT myself right now. So if I'm an enterprise, why do I need to be thinking about some operational organization around how my company uses things like ChatGPT or the like? Because if I can go do it on my own, why do I need other tools or help or anything like that?
Morgan Llewellyn: And it depends on the use case and how you want to use this type of product. If you're using ChatGPT to just query, " Hey, tell me about the history of X, Y, Z," you don't need that. But if you're putting it into a product, if you're putting it into a process, if you're really putting it into a business where business results are predicated upon that result being reliable and consistent, then you need a little bit more than just a call to a ChatGPT or an OpenAI or a LAMA. You need more than just a call to an LLM. You need some process and infrastructure in place to make sure the results that are being returned are consistent and reliable and really give your customer or whomever your end user is a great experience. Because if you give them a poor experience, you're going to lose them.
Zach Linder: What do you think is going to come next? So a year ago, we probably wouldn't have forecasted where we are today, which makes it even more hard to forecast where we're going to be in a year, but where are we going to be in a year?
Morgan Llewellyn: So I think a couple of things that we're going to see is we're going to see organizations, in particular probably on the data side, figure out how do you ingest all the documents you have in your organization or how do you ingest all the information in your organization and make it available to another lab? I think we're going to see that organizations are figuring that out. We're seeing that in the space now. And so the security, all of those concerns are going to be worked out and it'll be well contained within the cloud. But again, stressing out, they're going to figure out the data pipeline that makes all the information in that organization available to an LOM. So I think we're going to see that happen. And then the second thing I think we're going to see happen is there's going to be an organization that figures out how do you put that LOM across your entire data stack? So think of your SQL databases, think of your unstructured documents, your entire data link. Someone's going to figure out how to be able to make all that information queryable in a natural and intrinsic way so that your executives can query their business and get whatever pulse they want. And I was talking to a gentleman just recently and he gave a great use case of how does that improve someone's life. Imagine it's 4:30 and the CEO sends an email saying, " Hey, I need to know this number by Monday morning at 9: 00 AM." Well, now that CEO doesn't have to send an email and ask someone else to go do it, they could actually do it themselves at a drop of a hat. And so I think there's going to be A, this work on the data pipeline. And then B, you're going to see this holistic framework set up over that data lake or over your entire data architecture that allows you to query it in a natural way where anyone can get any question answered immediately, whether that be CSR, whether that be the CEO from the highest of those.
Zach Linder: I think we've all worked in organizations that have lots of content, right? The confluence pages, JIRA tickets, knowledge basis, case studies, a lot of it's very relevant and accurate. A lot of it is not. How concerned do I need to be? Can I just wholesale, put my knowledge base into the machine or do I need to cleanse that stuff first?
Morgan Llewellyn: So it depends a little bit on your use case, and I think there's-
Zach Linder: I want it to be accurate, right? I want right information.
Morgan Llewellyn: And I think that's the key, right? Coming back to this idea of consistency and reliability. And I think you also want to think a little bit about how do you make this feature proof? So you're at GPT, maybe you're using three and a half today or four, OpenAI three and a half or four, you're using LAMA two and another version comes out the next day and the next day. How do you make those models interchangeable without destroying your entire data stack is another question. And so if you really want to think about reliable consistent results, something that we think about is taking what we would call a RAG approach where you're essentially taking those documents and you're making them available for search, vector databases is another technology. And you essentially think about being able to take those documents and you're putting in a query and saying, " Hey, here are all the relevant documents that I'm interested in, now use an LLM to answer the question over that relevant document." And so that's what we see a lot of. We've implemented this for quite some time now. Actually, we were one of the first... You and I have been doing this for quite some time, funny enough, before it even existed as a thing, we've been implementing RAG. And so RAG is a really great approach if you need to bring in information about your organization and you need the results to be consistent, reliable, anchoring them in your own organization's documents is super helpful.
Zach Linder: So if we have a knowledge base that we want to go throw into a vector database, do we need to scan that to make sure that yes, this is accurate, this is in fact legit, or is that something that we can just machine handle that for us?
Morgan Llewellyn: So this is where I think depending on the size of your organization, the types of data that you're looking to put in there, if you were putting in publicly available documents, you can generate embeddings and throw them in. One thing you might want to think about is if these documents have sensitive information, if they have, again, some of the PHI, the PI. Now if you take that document and you generate the vettings, you've basically removed all the PI from it, at some level. But have you actually? And is it still okay to have PII embedded in that embedment? And I think that's a question that it's really got to be answered at the business use case. And so what you need to do to prep those documents for ingestion into a vector database is really use case dependent. And that's probably a first step that you should be considering when you're thinking about this journey.
Zach Linder: That's super helpful. You read a lot about how you might not know where the answer comes from, right? There's a black box, you ask a question, you get an answer. Maybe it's an actual answer, maybe it's factual, maybe it's hallucination, maybe it's somewhere in between. How do I stop the machine with my own private data? Can I get any auditability out of my answers to make sure that I know where this is coming from, what the source systems are? So if there is misinformation or an incorrect statement that I can go track that down and correct it in the source.
Morgan Llewellyn: Yeah. So what you can do if you take a RAG style approach, and let's say you feed it three documents and you have a question and you say, " Hey, I want to know what was the five locations of our stores in Minneapolis." And it'll come back and give you an answer, " Here are the five locations." And you can specifically ask it what documents or what information did you consider to give me this answer? And so it is possible, and I guess coming back to your excitement question, what is something that gets me super excited about something like an LLM is you can ask it, where did you get this information from? How did you come up with this answer? Whereas if you go back to something like some of these old neural nets, or even before that your standard machine learning, it was more of a black box. It's like, well, if you happen to have this income and you happen to be this gender, and if you happen to have bought these products, yada, yada, yada, then it generates a score. And that's more or less why we put in this bucket, right? With an LLM, it can be a little bit more prescriptive and transparent and accessible in it's understanding of, look, why did you tell me that these were the five store locations in Minneapolis? It'll say, " Well, we looked at the documents and these are the five leases that we have for Minneapolis," something like that.
Zach Linder: So through all of our conversation today, I'm hearing that there's a lot of opportunity for non- technical or semi- technical skills to go potentially implement, but definitely maintain a lot of these systems but it doesn't seem like there's a lot of deep technical knowledge. Data scientists were all the rage 10 years ago, and we need somebody that could go build theoretical models of whether this thing works or not, right? Let's go test that out. And I think now we're at the point where that's not the key skillset anymore. We're probably opening this capability up to a lot of different skill sets.
Morgan Llewellyn: Yes. I agree that you don't need a data scientist, at least not day one. What you really need is software engineers and someone on the strategy side who understands where do you want to go as an organization? What are the opportunities out there from a LLM generative AI perspective, how does it fit into my product and my target market? So you really need that more strategic and creative thought upfront, and that's what you need day one to implement inaudible. From a technical perspective, where you still have value in, I don't want to call it necessarily a data scientist, but someone on the analytic side side is what an LLM does is it's really good at generating content and it drives the cost of that content to zero. Anyone can go and generate a thousand recommendations almost instantly. The problem is, those recommendations have more or less zero value or even potentially negative value if you give the wrong recommendation to the wrong person. So I've gone and generated 100 recommendations and suggested this to you, but I told you, " Hey Zach," I know that you like black tea personally. I just know that's what you like. You like it still cold. Iced black tea. But if I'm using an LLM and it says, " Hey, here's 100 things that we could go give Zach, right?" We've got Sprite, we have Diet Coke, we have black iced tea, hot right? Earl Grey, hot Earl Grey, my favorite, but your ice tea is number 99 on the list, and we go and pick Earl Gray. Well, we've now given you a bad experience. And so the opportunity for the data scientists and the analytics folks in your organization is, how do you use your historical information? How do you use what you know about Zach to help focus the results of that LLM and make sure that you're providing great content to your end user? Because we are getting to a more personalized approach. And so now your value isn't the LLM, it has zero value because anyone can go and generate those recommendations. Anyone can go and generate that content. Anyone can go and generate automation. The value though, is the ability to exploit that automation, to exploit those recommendations and that content and be able to pair it with your historical information around what Zach's preferences are to make sure that you are recommending the right thing to Zach. That's the real value. And so you don't need a data scientist on day one. You don't need that analytics team on day one. You need some strategies, some forethought and some software engineering, but in order to really separate yourself from the competition, you're going to want to bring in that data science element because you're going to want to build predictive models on top of those recommendations to make sure you're always giving the right recommendation to the right person.
Zach Linder: There was a lot of focus on Zach there, and I feel like the machine was talking to me and I feel like it's preferenced to my likes, and I really appreciate that. So I think that's probably going to be about enough for us today. I just have one last question for you. If I were to look at your ChatGPT history, what's your last prompt?
Morgan Llewellyn: So I use ChatGPT almost as a replacement for Google at this point. Not if I'm looking for something recent, of course, but if I'm looking for something historical, I've actually used ChatGPT to tell me things about Google that I couldn't find on Google. It's just amazing. My last thing I looked at though was around finance and some different financial legislation around data privacy. That's what I used it for.
Zach Linder: Mine was how to give me a few questions to host a podcast about generative AI and LLM.
Morgan Llewellyn: And how did it do? How did it do?
Zach Linder: I used every single one of them. All right. Well, I appreciate it, Morgan, thanks so much for joining me today, and thanks everyone that listened. We appreciate it, and you'll hear more from us at Stellar Sessions.
DESCRIPTION
Casted presents conversations from Rally Innovations and Midwest House. In this session, Stellar's Chief Operating Officer Zach Linder and Chief Strategy Officer Morgan Llewellyn talk about their business and its goal to help organizations incorporate AI in their operations. Together they cover topics such as use cases for large language models (LLMs), when to jump into an evolving market, and the opportunities AI unleashes for internal and external end users.
Today's Guests
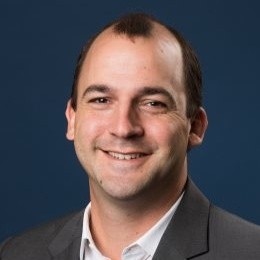
Morgan Llewellyn
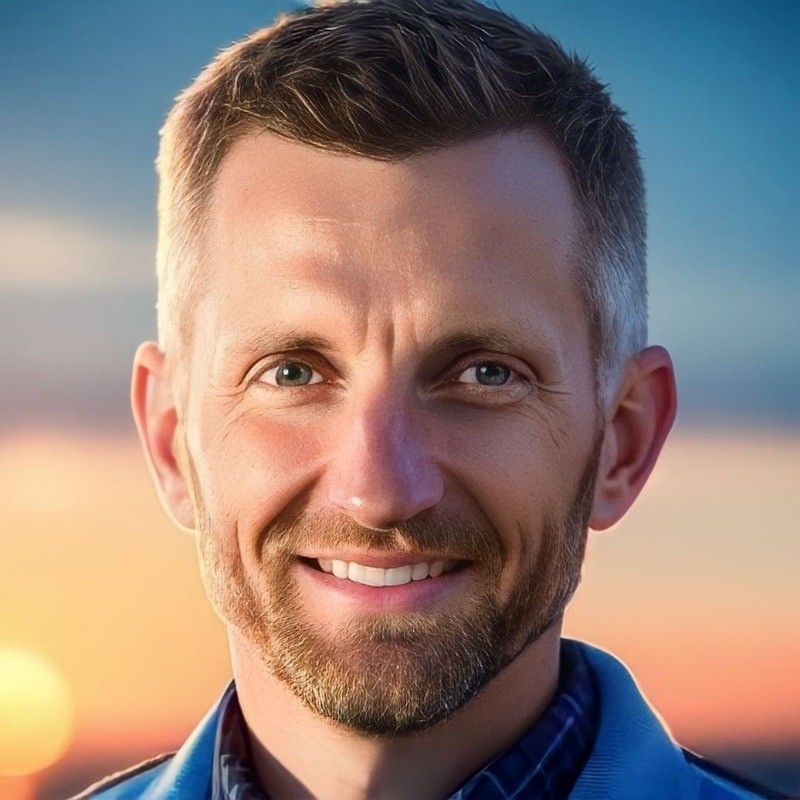